In case you're asking: why isn't this about tariffs? I've mentioned in the past that I don't think it's that useful for me to cover "breaking news." The situation is still quite fluid and I'm still seeing whiplash in even what certain provisions mean.
Next week or the week after, if things "stabilize" is probably a more appropriate time, especially to weigh in on the macro mixed with tech. In the meantime, it's still useful to consider what's a good endgame for foundational model companies in our brave new world.
Edit: I wrote the above before the 90-day non-China tariffs pause, which further makes my point.
I always talk about how most AI startups, especially foundational model companies, are likely doomed to flush their money down the toilet. I even did a panel on the topic recently at SXSW called “Most AI Startups Are Doomed” (and the recording is now up if you’d like to catch it).
There are reasons why I keep coming back to this theme.
There’s the boring reason, which is most startups die. Trivially true—and also not my core point.
There’s the obvious reason: AI is in a hype cycle and tech booms, almost as a rule, end in busts. That’s understandable, natural, and not necessarily a bad thing as I discussed in an earlier post.
But finally, there’s the real reason: these companies have no moat. I’ve explained before that there’s three core ingredients that go into AI:
Data—which for LLMs, everyone scraped the internet of content, with at times dubious legality and morality
Models—which are all based on the transformer architecture. Not to diminish the incredible work in fine-tuning, post-training, and innovations like Mixture-of-Experts (which some of the latest models, like DeepSeek v3 and the just-released Llama 4 are). But the 80/20 is still largely the same and new techniques diffuse to everyone.
Compute—everyone uses NVIDIA GPUs (even DeepSeek), which I explained more qualitatively why before, but the computational layer is basically all shared. xAI caught up by just throwing money at the problem and buying 100,000 of them.
With billions of dollars of investment in foundational companies that are largely the same, there are bound to be many spectacular busts.
So if these companies can’t win on differentiated product, and they can’t win on defensibility, what’s left? That’s what I’d like to focus on today.
There is one—and only one—real success case I can see.
At least, only one where we put aside the hard AI takeoff, Superintelligence, God-in-a-box AGI dream with the first company that achieves it hitting the “I win” button forever. (In which case, for humans, do we really win?)
No, the real path to winning would be this:
Make frontier model for ridiculous amounts of money.
Have frontier model get commoditized (including by open weight model).
Most importantly: Run out of customers (?!)
In other words: become a natural monopoly. Winner takes all.
Quick Background on Natural Monopolies
How do natural monopolies work? I’ll skip the full Econ 101 and try to provide the intuition for it.
In essence, it must be too expensive/unprofitable for more than one (or maybe two) players to exist. Basically, the elements are:
Huge fixed costs—making it very expensive to even start playing the game.
Relatively low marginal costs—each customer doesn’t cost that much more to serve. In other words, economies of scale and bigger-is-better.
Finite, exhaustible demand—because otherwise, effectively infinite demand means there’s more space for more players.
(You can also have a natural oligopoly that works largely similarly with a couple of players, instead of 1-2 players, but I’ll simplify to natural monopoly.)
If these conditions are achieved, no one else will want to enter, giving you the ability to raise prices and enjoy the spoils (economic rents/excess profits) of owning a monopoly.
Traditional utilities are the classic example in economics. It’s very expensive to set them up, it’s relatively cheap to service every single customer, but there are only so many customers in a geographic area for power/water/etc.
Google, Meta, OpenAI, Anthropic, and others are already spending a lot of money on developing foundational models, but in the natural monopoly scenario it needs to cost even more and the market needs to be completely saturated.
There’s some evidence we’re going in that direction.
Training/hardware/fixed costs for new frontier models are exponentially increasing.
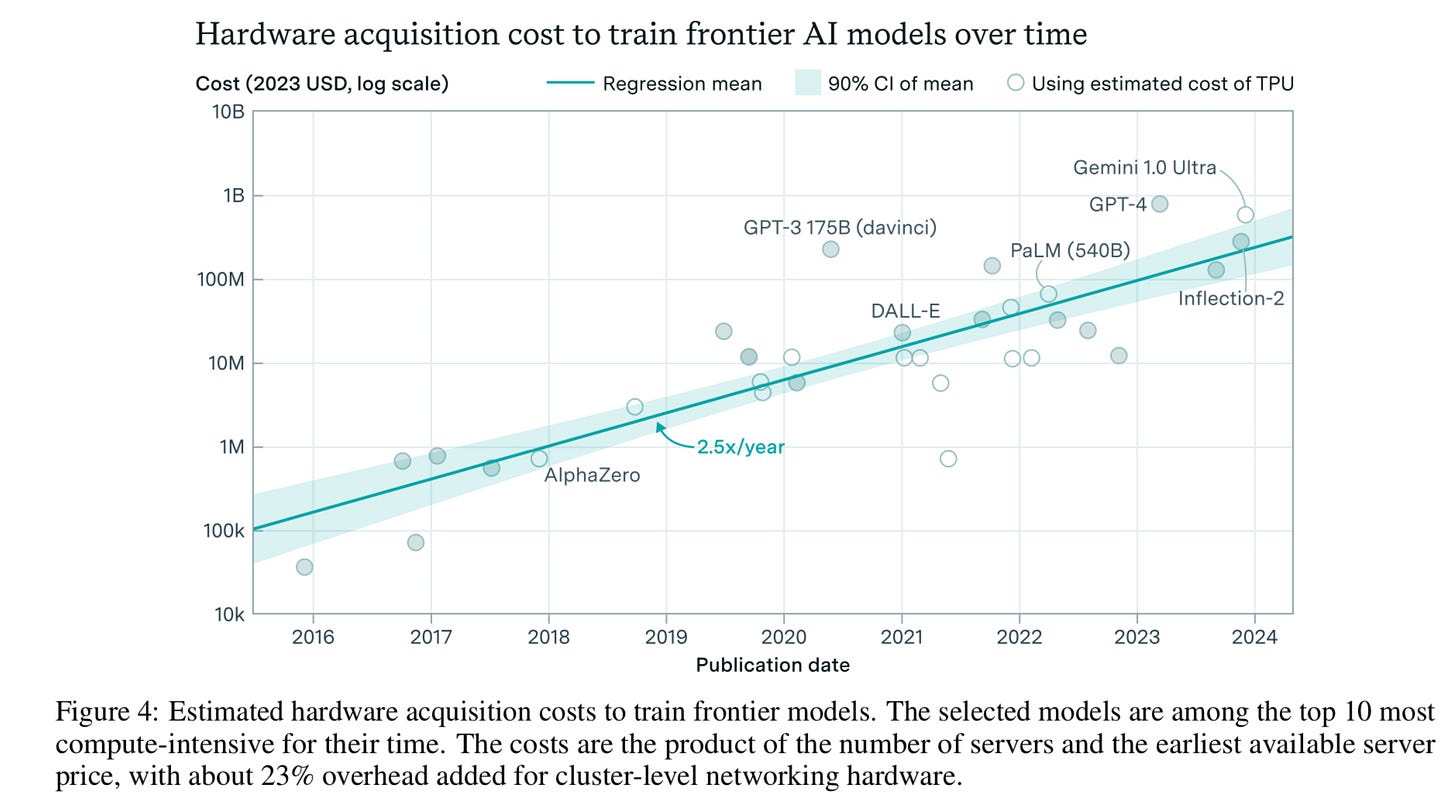
Inference costs are exponentially falling.
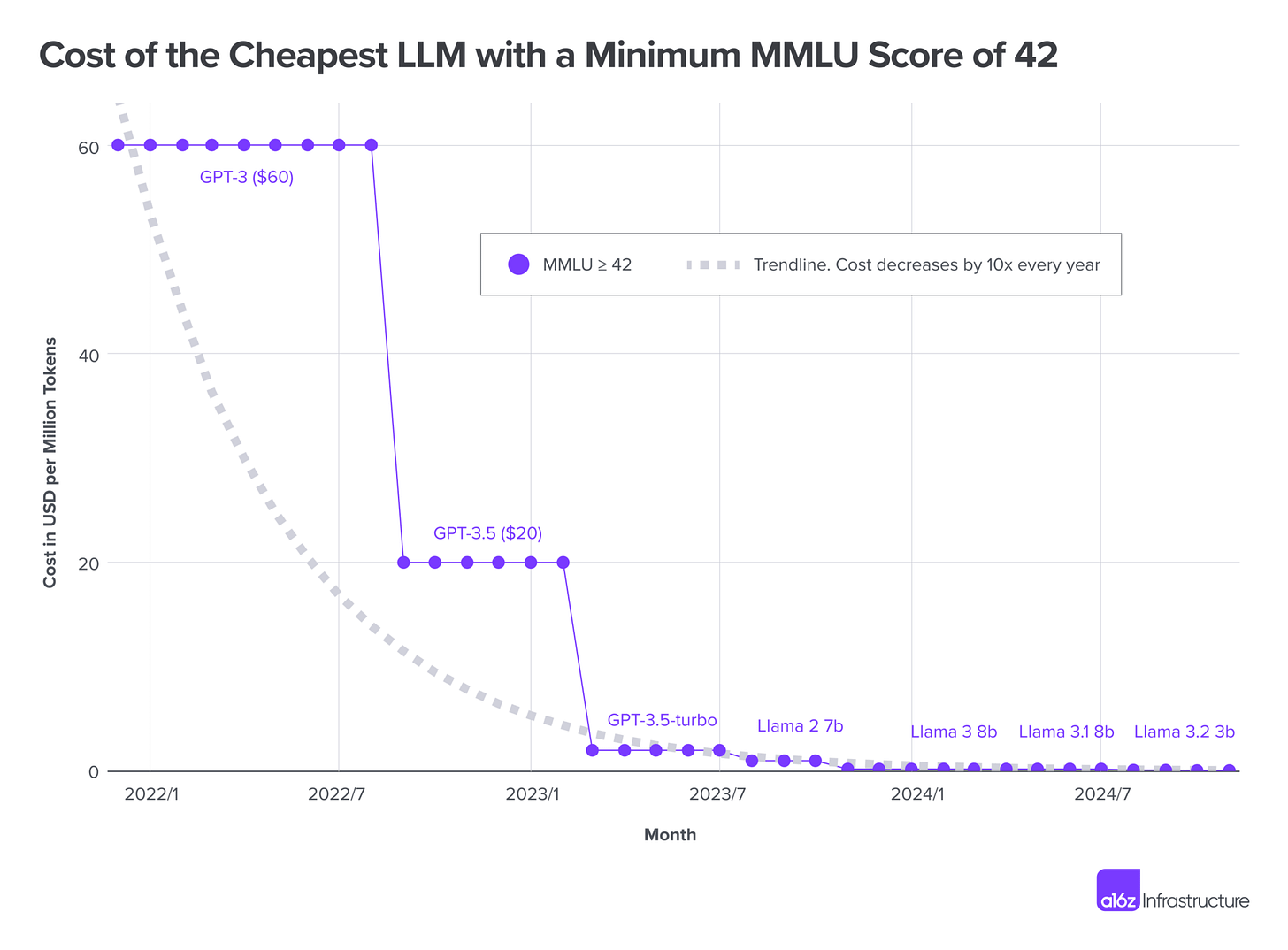
A lot of people are already using AI. OpenAI has over 400 million weekly active users as of February 2025 and is targeting one billion by the end of 2025. All of Meta’s products (Instagram, WhatsApp, Facebook, etc.) have around 4 billion monthly active users. It’s not quite saturated, but it’s not a few techies using ChatGPT and similar anymore.
But this is all theoretical and utilities are kind of boring. This hasn’t happened within tech, right?
TSMC Playbook: Lose Money, Own the Future
Back in the day, everyone (in semiconductors) had their own fab. Jerry Sanders, AMD’s founder, infamously jeered, “Real men have fabs.”
Morris Chang, founder of TSMC, understood something fundamental that others did not. Cutting-edge fabs were getting exponentially more expensive. Morris Chang started his career etching out chips by hand, then with projector screens. Years later, EUV is working with a fraction of the wavelength of visible light—and work by shooting tiny droplets of tin with lasers that somehow precisely deposit light to create modern chips. We’re almost at the point of pushing around atoms.
Given the exponential cost curves involved but low cost to create chips once the fab is created, it’s a natural monopoly. One fab should service Apple, AMD, NVIDIA—everyone. And that’s exactly what happened.
Intel’s fumble is that they owned their own fabs but did not amortize their costs well by servicing others. They missed the boat on mobile, but more critically missed the opportunity to compete with TSMC—Intel was famously arrogant and obnoxious to work with, compared with TSMC’s excellent customer service (and deliberate choice to not compete directly with their customers).
Is AI is heading that way? Maybe.
I showed a chart on training costs already, but let’s put words to it. Training GPT-4 reportedly cost over $100 million. Gemini Ultra? More like $200M. Anthropic has already said they expect to spend billions on Claude’s next iterations. Elon Musk's xAI has built a 100,000 GPU supercluster for Grok 3. And Google plans to spend $75 billion this year alone — mostly for AI infrastructure.
It’s the classic “high fixed cost, low marginal cost” business model. It looks terrible at first. You sink hundreds of millions into model training, data wrangling, and GPU clusters. But once the model is trained, inference becomes cheap—which didn’t used to be the case/wasn’t certain, but now seems clear.
So you need to serve billions of queries to make your money back.
Which brings us to the actual trick: once you build it, you need to serve everyone. Literally everyone. Which often isn’t guaranteed.
What else could happen?
We could do a lot more inference off the cloud and on the edge. Although Apple’s AI attempts have sucked, their hardware is great and we could see small models run on-device for “free.” After all, if LLMs become “good enough” and benefits from new models plateau, it may be easier just to run local models with our much more powerful AI chips in 3-5 years.
While that could be good (marginal cost is even lower, i.e., free for the model company), that scenario probably also means that the value to being at the absolute frontier is much lower. Competition over being the “cutting edge fab”—in this case, frontier model—would be much lower, which means those massive entry costs would be less. These model-makers would likely compete off of other things: like customer brand recognition, which OpenAI’s ChatGPT is by far in the lead for right now. But brand isn’t really a stable or particularly powerful barrier and limits your pricing power.
Alternatively, instead of costs rising, we could see training costs also radically fall alongside inference costs with better techniques, basically doing the opposite of what’s needed.
Finally, demand could expand too much, which is ironic. Again, that might sound good—more customers!—but it also brings in more entrants and prevents a natural monopoly.
That latter scenario is also “ok” (it means the model companies are probably making money), but they aren’t going to make so much that they can likely justify their sky-high investments and valuations. Basically, the foundational model companies would likely survive, but would likely lose their investors money. Which, honestly, has been most of what I’m saying: not that all of them will die, but they won’t justify their investments (and some/a lot of them will die).
What do I think will happen?
It’s hard to say. My personal take is we’re still in the early game of AI.
However, it’s important to note that there’s a lot of ways that I described where things can go wrong. It isn’t like it’s a nice linear path to a natural monopoly for foundational model companies.
It’s quite plausible that some of those things like the rise of edge models, competition on other factors, etc. become central to the AI industry.
Besides that, in order to play this game at all, you need to dump in billions—potentially, collectively trillions—on the hope that this is the way it goes. Again, going back to my bubbles article, this is how things do generally work in tech booms, but that’s cold comfort for investors who lose a ton of money from it. (But, as I mention there, it’s great for us as society, since we then get a lot of goodies privately financed)
Finally, remember something about natural monopolies like utilities: they generally get regulated. We’ve already seen a lot of global conversation about regulating AI. If they do achieve natural monopoly status, that’s going to just accelerate if not make it guaranteed. Achieving this “win condition” might be a poisoned challis.
Nonetheless, Microsoft didn’t exactly cry over becoming a monopoly and “broken up” (poorly) by the US government. But utilities are traditionally not great businesses and are much more common examples than Microsoft.
What will happen? I guess we’ll see. But this is my framework for thinking about “success cases” and how foundational model companies can (maybe) justify their valuations and investment.
Thanks for reading!
I hope you enjoyed this article. If you’d like to learn more about AI’s past, present, and future in an easy to understand way, I’m working on a book titled What You Need to Know About AI that will be published later this year.
You can check out a sneak peek of the introduction and first chapter here!
Would love to hear your thoughts on this -
https://artificialintelligencemadesimple.substack.com/p/how-will-foundation-models-make-money?utm_source=publication-search
Really enjoyed this – especially the natural monopoly angle and the TSMC parallel.
Switzerland’s playing a totally different AI game: decentralized, regulation-forward, lots of research–industry crossover. No billion-dollar burn, but it still works.
We broke it down from the Greater Zurich angle – no scale race, but still real traction:
👉 The Big Swiss AI Secret : https://www.greaterzuricharea.com/en/news/big-swiss-ai-secret-how-small-country-challenges-tech-giants
Substack: https://open.substack.com/pub/greaterzuricharea/p/the-big-swiss-ai-secret-why-tech?r=3g6z3y&utm_campaign=post&utm_medium=web