An analog in semiconductors
Intel has been struggling for years, but the recent firing of CEO Pat Gelsinger, the failure of his “IDM 2.0” (Integrated Device Manufacturer), and serious challenges faced by the company have spurred reflection on my part about its history.
From its founding from the “Traitorous Eight” leaving Fairchild Semiconductor, to its early innovations in silicon gate technology, to its role in early memory, and then to logic, and its “Tick-Tock” strategy of pushing forward semiconductor performance, and, of course, its invention of Moore’s Law… Intel has stood in the center of making the modern exponential scaling of computing a reality.
FinFETs, backplane power, ribbonFET, and a whole pile of innovations (which, if you’re interested, you should read folks like Babbage at The Chip Letter), Moore’s Law of “transistors in an IC doubling every two years” was not automatic by any means.
It was an act of will, human creativity, and billions of dollars. And while I don’t want to diminish the contributions of others—TSMC has its technologies, and Samsung has been no slouch—Intel has, for years, been the vanguard forcing semiconductor technology to advance at a blistering pace.
Moore’s Law was a business strategy. It was a choice.
And it’s still yet to be seen whether the other companies will truly pick up the mantle that Intel has now vacated.1
Scaling in AI
There’s been a bunch of takes that I suggest about the topic that you should read for the technical nuances, my point here is not to debate “is scaling dead,” or not.
My point is scaling is a choice, and it’ll happen if the players in the market want it to happen, and it won’t if they don’t.
Because the point of the analog of semiconductors is that Moore’s Law was not a Photoshop “image scale” of the transistors to make them 50% the size or something. After a certain point (and not even that late in the game), there had to be really clever engineering to put in different types of structures, build up from 2D to 3D, and a ton of other really hard innovations.
Even in AI, if you’ve been paying attention to what the companies have been stating publicly, have not been merely, “Throw more compute and data at it.”
I like Nathan Lambert’s “A recipe for frontier model post-training” for this—it really helps illustrate how much spaghetti is being thrown at the wall to try to improve things because if it takes all of these varied methods to help make frontier models a reality, think about how much didn’t work.
That sounds derogatory, but that’s honestly just the R&D process: you don’t know it doesn’t work until… well, it doesn’t work.
As such, to repeat myself, scaling is a choice.
A pet peeve
This has been a constant refrain on my side: yes, sheer compute, power, and a bunch of stuff in terms of resources is important for training powerful models. It’s constantly what I hear about in rooms full of AI entrepreneurs and VCs.
I tend to be the weird voice constantly saying that, “AI’s big leaps have come from better models—doing more with less.”
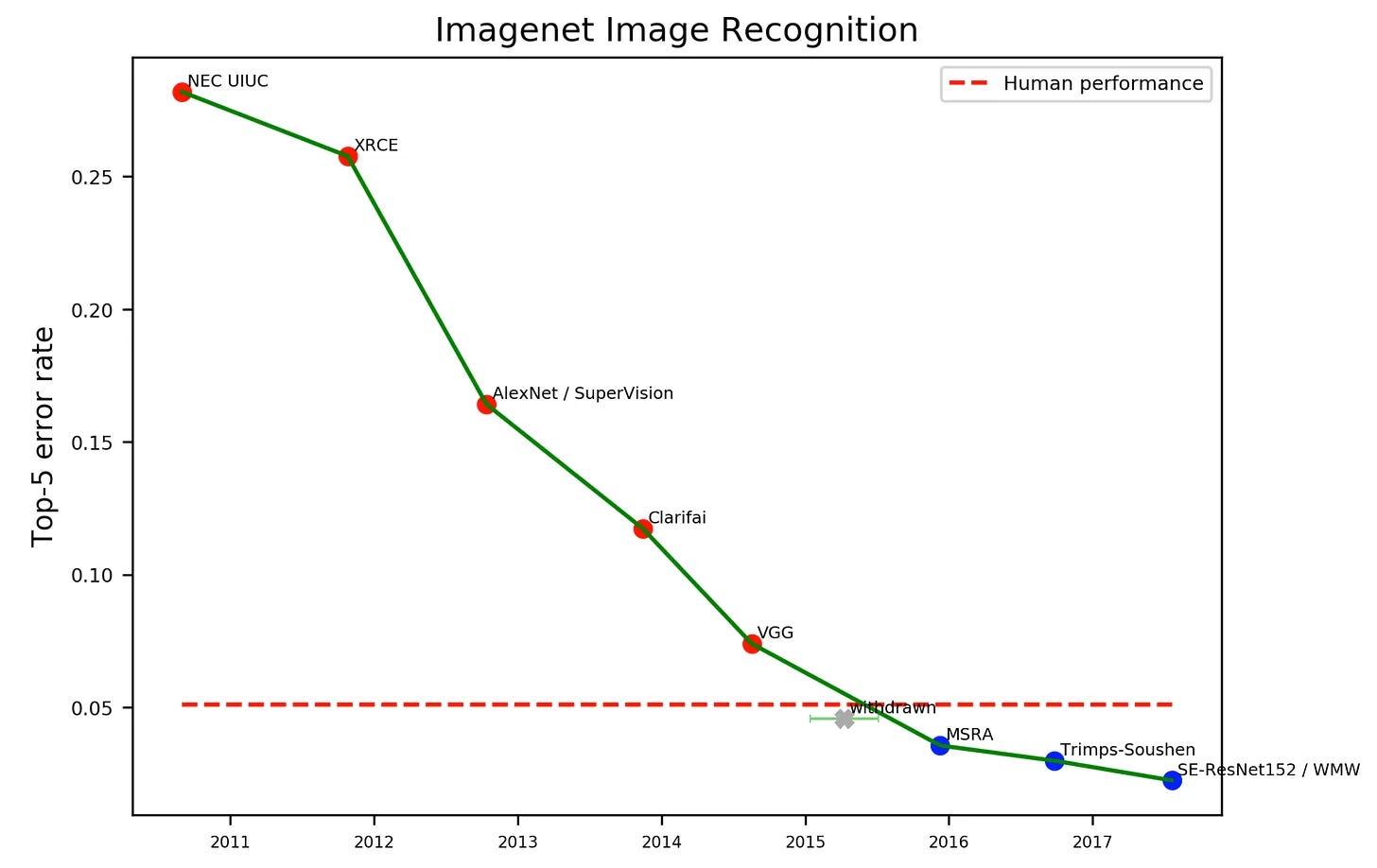
Not to appeal to authority, but it’s just too good to pass up given OpenAI has dumped a huge number of emails between Elon Musk and the OpenAI team. I want to call attention, in particular, to Ilya Sutskever’s emails (for context, he is the co-founder, former Chief Scientist at OpenAI, and one of the authors of AlexNet one of the early triumphs in the ImageNet benchmark).
Quotes pulled out with emphasis mine.
We can’t build AI today because we lack key ideas (computers may be too slow, too, but we can’t tell). Powerful ideas are produced by top people. Massive clusters help, and are very worth getting, but they play a less important role.
Additionally, talking about compute:
Compute is used in two ways: it is used to run a big experiment quickly, and it is used to run many experiments in parallel. 95% of progress comes from the ability to run big experiments quickly… For this reason, an academic lab could compete with Google, because Google’s only advantage was the ability to run many experiments.
The point here is the power of having a lot of compute wasn’t considered a massive advantage just because it was a lot of compute. It gave the ability to experiment and iterate quickly. As startup founders know, this is one of the ways that the best companies win: they just try more stuff, figure things out, and then blow past their competition.
Scaling is a choice. In this case, scaling—as defined by radically increasing performance with more resources—is not just more compute, but actually more innovation. It doesn’t just happen.
This is the same thing that happened with various innovators in semiconductors—not restricted to, but largely led by, Intel.
Other implications, especially for AGI
All of this put together means that a massive amount of time, money, and resources will be required for R&D to keep pushing forward AI.
It also means that we won’t necessarily stay on the same crazy curve where AI consumes all of our resources, despite Meta seeking to build a 4GW nuclear plant and Microsoft seeking to restart Three Mile Island.
It’s just really hard to say what the future modality of AI is. Is it likely to take a lot of power? Yeah, probably, but the kind of apocalyptically high-power requirements we’re speculating about? Hard to say.
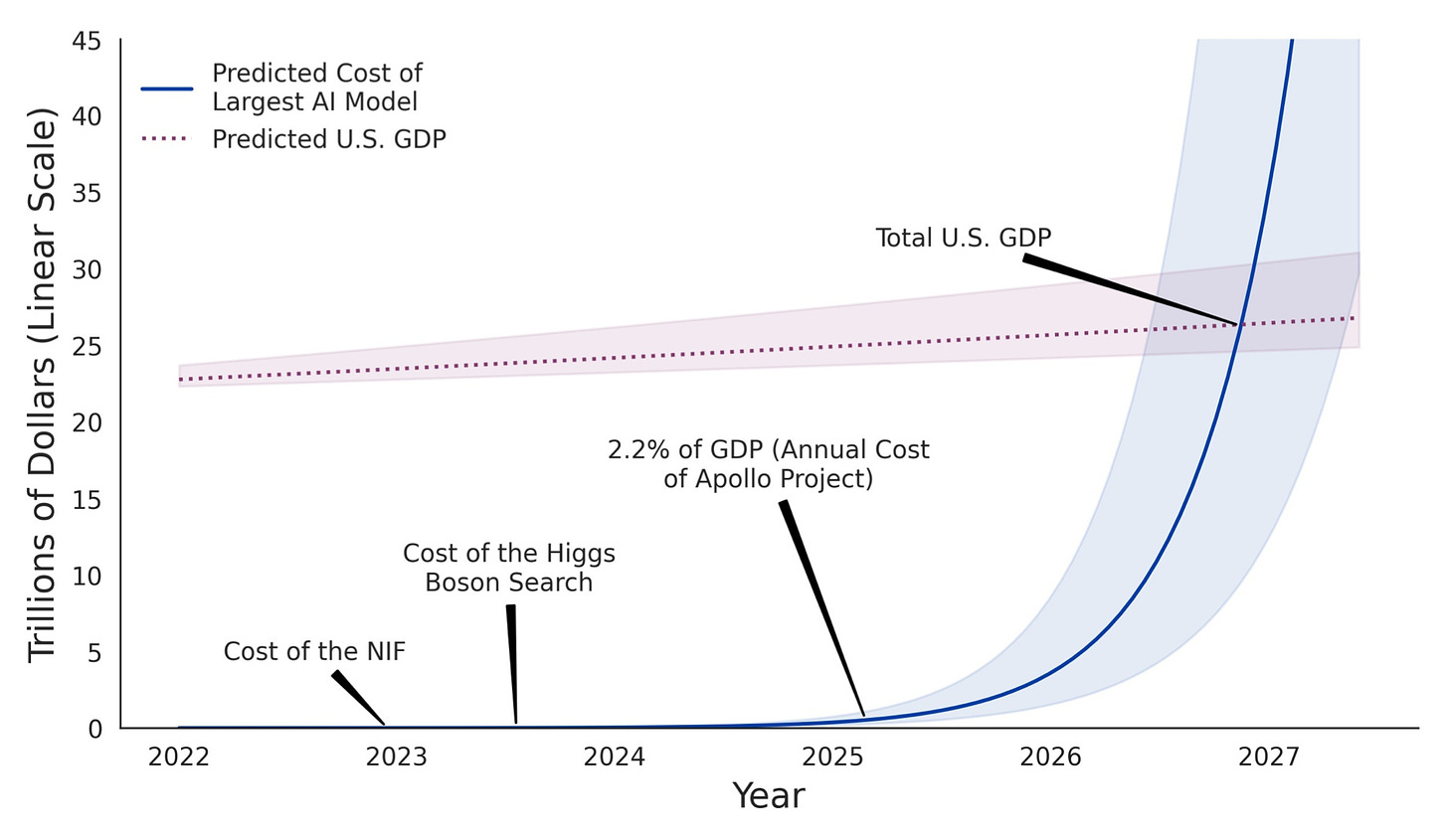
This all also means that I find a hard takeoff scenario for AGI (Artificial General Intelligence) hard-to-believe.
Here, I define AGI as “human-like” intelligence, with all of our creativity, reasoning, and “human” abilities—despite organizations like OpenAI recently trying to weaken the definition (perhaps, cynically, as a negotiating tactic by weaponing the AGI clause they have with Microsoft).
But regardless, AGI doing a “hard takeoff” requires a huge amount of work and creativity that AI fundamentally hasn’t shown. And is unlikely to show, given deep learning AI, definitionally, learns a statistical distribution from data… and doesn’t have a plausible way to suddenly “break out” of the distribution and do creative work outside of it. And that’s precisely what’s needed to keep scaling.
Final implications
So what’s the takeaway? Well, to see advancements, we’re going to need to continue to see investment, smart people, and creative solutions from the leading AI labs of today.
It will be model enhancements and advancements. We aren’t going to magically get scaling from just throwing more compute and data at stuff (because, even today, we have to do a fair amount of tuning on the data side, let alone the model side).
And, of course, the AI future will be unpredictable. Because, by definition, what we discover will be a result of R&D and creativity, not just a straight-line extrapolation from what we have today—no matter how much like a straight-line, “image scale” function, or inevitable process it may look like from the outside.
Thanks for reading!
I hope you enjoyed this article. If you’d like to learn more about AI’s past, present, and future in an easy to understand way, I’m working on a book titled What You Need to Know About AI that will be published in 2025.
Sign up below to get updates on the book’s development and release here!
Perhaps it’s self-delusion, but I still hold out some hope for Intel, and not just because I hold its stock in my public market portfolio…