In the process of researching and writing my book What You Need To Know About AI, I’ve had some great conversations with experts with unique, first hand perspectives on AI. I’ll be sharing some of these interviews here because I think some of you will enjoy reading them in full, and it’ll give a taste of what’s to come in the book.
If you’d like to get updates on the book’s development and release, sign up below:
The first interview I’ll be publishing is with IMVARIA. IMVARIA brings artificial intelligence to imaging and lab data, with non-invasive biomarkers that can completely revolutionize clinical assessments in serious diseases. Their first product is in market: FIBRESOLVE, the first FDA-authorized AI diagnostic tool for ILD (interstitial lung disease) and IPF (idiopathic pulmonary fibrosis).
(Full Disclosure: Creative Ventures is an investor in the company)
The founders, Dr. Joshua Reicher and Dr. Michael Muelly, are also both medical doctors and faculty at Stanford Health Care in Radiology. They also both worked at Google on AI and have numerous publications on it in prominent machine learning and AI journals. They have a rare perspective, sitting on "both sides of the table" between medicine and technology.
You can read the transcript below (edited for clarity) or listen to our conversation above.
Topics Discussed
How did you become interested in AI and Medicine?
What is the biggest impact AI could have in medicine in terms of clinical care for patients?
What has changed in AI to make these things now possible?
What are AI’s greatest weaknesses in medicine right now?
What is your response to “deep learning is a black box”?
Should humans be in the loop when working with AI in medical applications?
Thoughts about Strawberry o1 results (for medical diagnosis)
What is IMVARIA?
How would you talk to other clinicians about AI and medicine?
Why do you think radiology in particular has been resistant to adopting AI?
What does a radiologist actually do?
What has been the problem with clinical decision tools in the past?
Do you think EMR data could become a big deal within AI and healthcare?
What should not be replaced in AI and medicine in the near term?
Will OpenAI and other foundational model companies take over in medicine?
Why won’t China be the leaders in AI and healthcare?
Impact of simulated or synthetic data in healthcare
Final thoughts
How did you become interested in AI and Medicine?
James Wang:
What made you both interested in AI and medicine in the first place?
Michael Muelly:
For me, it was not so much AI specifically, but really I wanted to apply computer science to medicine. I started out in the computer science side. I did network, like working in network security for a couple of years after high school. I worked with a couple of banks in Switzerland and yeah, really that was kind of boring in the end. Dealing with all the financial stuff, right?
I met my wife, who's American, so then I moved to the US and really studied computer science then and entered medical school with the goal of applying computer science to medicine. So for me, it all goes back to that.
And AI is like the great area for application in medicine today, right? We're like past making the US healthcare system digital, but now it's actually about the applications and AI is the big piece there.
James Wang:
Josh, anything on your side?
Joshua Reicher:
Yeah. For me, Michael's history goes a lot farther back on the sort of artificial intelligence side.
But for me, basically, I was interested in software as a kid. I built a number of different coding applications over the years and then what I really enjoyed doing for a long time was building technology that would allow doctors, clinicians, people working in hospitals and clinics to do their jobs better, right? Be more efficient and more organized, better patient communication, those sorts of things.
After that while I was spending some time on the investing side I started to see that there's really kind of two worlds in medicine because there's how do you make the system work better, but in the end, there's still how do you actually make patients' lives better? And sometimes, actually, I would say a lot of times, that actually gets lost, right? There's a lot of technology that's developed actually to make the doctor's life better.
And I had a mentor who was a cardiologist who at one point was talking about the use of anticoagulation and taking patients on and off of that perioperatively. And he sort of highlighted that even stuff that sounds very clinical, a lot of times actually driven by just making the doctor's life easier, like less bleeding during a procedure, like stuff like that.
Michael Muelly:
The DaVinci robot is like that, right?
Joshua Reicher:
Exactly.
Michael Muelly:
There's very little data for like the prostate, radical prostatectomies, but then people still do it because it's better for the surgeon, right?
Joshua Reicher:
So what for me kind of came together is then at around the same time Michael and I were starting to do research in AI was the results from the scientific work that to me connoted something that actually was gonna be impactful on a patient level, like actually make a meaningful clinical impact in terms of outcomes.
And that was where I started to pivot a lot of my interest in work into saying like, no, this is an entirely new category. And that's why we ended up going into doing something that was more clinically directed and not let's say, AI for billing or something like that.
What is the biggest impact AI could have in medicine in terms of clinical care for patients?
James Wang:
Right. And I mean, you're getting to it, but maybe what do you think is the biggest potential theoretical area of greatest impact that AI can have within medicine, within clinical care for the patients?
Michael Muelly:
I think actually creating the different groups of diseases, right, that actually respond to treatments best. So being able to target the treatments to specific groups of patients, I think is one of the biggest potentials, right? Because a lot of the diseases that we diagnose are very heterogeneous and being able to bettersubcharacterize this disease, this disease state phenotype is gonna respond to this drug. It's gonna be a lot easier then.
And I think that's probably one of the biggest things that we can do with AI, except for actually patient motivation. Motivating the patient to do things could potentially be done pretty well with AI.
James Wang:
Right, like patient compliance.
Michael Muelly:
And that is always something physicians struggle on.
James Wang:
Right, right.
Joshua Reicher:
Yeah, I agree. I mean, I think in the long-term view, obviously there's a lot of sort of fear around the use of AI, that sort of thing, but I think that ultimately the ability for these technologies to review and parse just vast amounts of data and do it very quickly is something that gets beyond human capacity and I think can have a deep and meaningful outcomes improvement.
I mean, there's a great study that came out earlier this year that showed the use of inpatient cardiac telemetry, which if you've worked ever in a hospital, there's literally like a room of technologists sitting there that looks like Minority Report or something with all the little like pacer leads for all the patients all through the hospital.
That doesn't actually make sense, because you're trying to follow potentially 20 different screens at the same time. It's sort of crazy that we still do things this way.
And there are so many applications like this where it is absolutely sensible and logical to automate the ability to do that. And we just haven't had the capacity or technology to do it before. So that's where I think there's just entirely new avenues of things that we really cannot do today, that this is already starting to enable and we've seen outcomes improvements for patients as well.
What changed in AI to make these things possible?
James Wang:
So what do you think is the biggest change? Like is it the models, is it the computing power, is it the data?
Joshua Reicher:
Yeah.
Michael Muelly:
Yeah, I mean, I think for us, and yeah, it's the computer vision side, right? And the computer vision side, it was really the statistical machine learning approach that they were using for a long time, which doesn't really work as well for visual features, which is ultimately what any radiological image is going to contain, needs to be analyzed. And so that really was the big leap forward going from just the statistical machine learning methods to deep learning and CNNs there.
Joshua Reicher:
I'm going to break the interview barrier here a little bit and just show it. This is the slide we show when we do some educational stuff, which is this sort of 2009, you know, era revolution.
I think that sort of spurred a lot of the work and obviously we've had additional steps forward here the last few years. But I mean, this to me is the core, right?
You had a combination of a data corpus and some major hardware advances that occurred at the same time that took stuff that had been theoretically possible for many, many decades, arguably longer than that, and actually make it possible.
Michael Muelly:
Yeah.
James Wang:
I love the ImageNet stuff. I've been trying to get a hold of Fei-Fei Li, but I think she's kind of busy right now.
Joshua Reicher:
So many people always try to get a hold of Fei-Fei, that's for sure.
James Wang:
Yeah. I mean, it's one of those things where she has plenty of public interviews and stuff, so I think that's probably fine. But yeah, I have ImageNet as also part of the personal story for when I got particularly interested in coming back to Silicon Valley and AI.
What are AI’s greatest weaknesses in medicine right now?
James Wang:
But yeah, maybe in terms of jumping to I think one of the things that you were referring to, Josh, well, actually both of you alluded to—there's a lot of fear about what AI can and can't do, and there are a lot of worries in terms of it. I'll jump into radiology specifically in a bit, but what do you have broadly to say to that?
What do you think are the greatest weaknesses in it, and how do you ensure safety for within this particular area?
Michael Muelly:
I mean, any, you know, optimization problem you can overfit, right? So there's always a problem with deep learning models that you have to guard against to make sure that that's not what's happening. You're overfitting to that specific data set, but you want it to generalize and apply to more general examples of the same disease. So you've got to then really collect a large training set range, right?
So you actually have a broad input that covers most of those cases. And then beyond that, you know, we do have some tests in place that make sure that the sample that you actually look at is within the distribution of what the training data actually contains, right?
That's really, on the technical side, how we safeguard. And then there's like the statistical, you know, look at it in clinical trials.
Josh, I don't know if you want to add some more pieces there.
Joshua Reicher:
Yeah, I mean, I think for clinical practice, our approach is to say broadly you should think about this the same way that you think about any medical technology, which is what is the use case, what is the risk benefit, you know? And how do you make that calculation? For us, we worked in diagnostics, and in diagnostics, there's a pretty straightforward sort of process for that in terms of clinical validation.
We worry a lot about generalizability. I think that's probably the one thing that maybe stands out a little bit more in AI relative to other types of clinical technologies. With that said, honestly, I've seen that even in just like standard diagnostics, too. You know, like build a lab test that works at one hospital [but] doesn't work at the next one.
In some ways [this problem is] less new than people maybe want to sort of see. But I do think proving generalizability by showing, you know, this technology can work in a lot of facilities and sites and patient groups, that sort of thing is very, very important.
And the FDA has made that very clear in, you know, extended work the last few years that they want to see that generalizability. So I think that's the biggest thing.
And then in terms of AI more broadly, I think it's in a lot of ways like any new technology, it's a combination between figuring out the position, but also the users.
And I think a lot of the fear is built, frankly, off of science fiction, which is people watching like I, Robot or The Terminator or something, and then imagining what AI means.
So, you know, a lot of times I like to say, like, we don't really love AI as a term because it invokes like a lot of that science fiction. And I think if you treat this for what it is, which is, you know, heavily mathematicalcomputational systems to help optimize decision making, not quite as sexy, but that's really, I think, a more accurate description.
Michael Muelly:
Yeah, and I think that the media reinforces that narrative, right? It's like, oh, we're going to get rid of radiologists by 2025, right?
And yeah, that makes it even harder with the media reinforcing that same narrative — and I think some investors, for example, actually going to invest because of that narrative, right? As opposed to the underlying company.
Joshua Reicher:
I think, too, comparative results are important. So like one thing that's been brought up a lot has been the concern for things like racial bias in AI, right?
And there's been demonstration of that and, you know, not just medicine, right? A broad range of different things.
But it's always important not to look at that in isolation. It's to look at it in comparison to what the current system is and can you improve upon it?
Because a lot of times we've seen things where it's like, actually, the AI system is not perfect and may still have these biases, but maybe substantially better than the current system.
And it's just all of that has to be taken with care.
Michael Muelly:
Right.
James Wang:
Yeah. I was about to say it's I would not say that current data sets and systems and trials are particularly racially or sex based balanced in terms of our understanding.
Joshua Reicher:
I mean, the FDA just published this was about a month ago, a new draft guidance that's literally around diversity in clinical trials. And, you know, with all the right, I think, intentions and also raises all sorts of challenges in particular to me, this is relevant in AI, because the reality is AI requires large data sets to do training.
The data sets from the last 20, 50 years are what they are. All you can do is hope to change things moving forward.
So there are some big challenges there.
What is your response to “deep learning is a black box”?
James Wang:
Right. I mean, speaking towards one of the things that you mentioned, deep learning, just whatever it is in terms of modern deep learning is a black box. And, you know, you've had historical criticisms of that from Marvin Minsky and a bunch of other folks.
And this is why MYCIN, which famously was not particularly generalizable in terms of expert systems, failed.
But yeah, you were talking about the statistics and whatnot, but what would be your thought in terms of response to that given, yeah, it's a black box, we can't audit it, you can't have any transparency in it, we should have knowledge based systems or symbolic based systems that were more provable and deterministic instead.
Michael Muelly:
I mean, that's our standard of care right now, right? As humans, where we don't actually know how did they form their views, right? How did they come to their diagnosis?
So it needs to be validated the same way that you would validate a human interpreter, right, for a specific disease. And do this in a statistical manner, similar to what a pharmaceutical would have to undergo.
And then if you've proven that you have the robustness, how is it different from somebody actually interpreting the data was trained to do so?
Joshua Reicher:
I think one of the things I really enjoyed working at Google was actually having extended kind of lunchtime conversations on this topic, because it's relevant to everybody. And I would describe myself essentially as a convert, because I think I was really skeptical of these sort of black box technologies.
And I think once you understand how they work and how they can go wrong, I think the concerns around performance issues sort of go away. So there are these challenges, which is like, if you get overall better performance, but you get different types of errors, then how do you account for those changes?
And the answer is like, you learn what those problems are, and then you account for them. And I think that just has to be taken on a kind of a one-by-one basis.
So personally, I think there are certain use cases that I don't think the current era and iteration of artificial intelligence technology are really capable of doing.
I as a physician would not probably trust a chatbot to talk to my patients on my behalf, right?
There's just certain things that like, I don't think we're there yet. The types of errors that can happen to me are too risky, relative to the potential benefit.
But there are a lot of use cases where that's not the case.
And then the other thing is, I think, education for users of these technologies for what are the errors that these technologies tend to make, and how can you look out for them and account for them and potentially correct for them? And we've seen that too.
What I see is that sometimes the errors that they make are, they make far fewer errors in what I would consider tricky situations, right? What's sort of hard for humans. And sometimes the errors they make are completely illogical, random things that you would never expect.
But it turns out humans are pretty good at sorting those out. And that's where I think the complementarity is most valuable.
Should humans be in the loop when working with AI in medical applications?
James Wang:
So would you say that you feel like there still should be humans in the loop, generally speaking, always?Where do you put the balance on that?
Michael Muelly:
I think you wouldn't want a machine learning model to run on a piece of data without right now having somebody look at it. But it really depends on how you build it into the workflow.
For our test, for example, the physician, we ultimately make sure that the result and the diagnosis correspond. So there is a human in the loop still.
Joshua Reicher:
Michael, what do you think about the current iteration of self-driving cars?
Michael Muelly:
Well, as long as they work, right?
Michael Muelly:
I guess in San Francisco, I don't know, I've had some issues standing behind them. They need to get the actual manual operator involved, right, a lot of times. So yeah, we're not quite there yet in terms of AI technology to completely solve all those edge cases.
Joshua Reicher:
Yeah, I think that's right. I think it's like, in general, what they call in the lab world, there's, I think they call it specific supervision and general supervision.
So specific supervision is, and I may be misusing the terms, but it's basically like every single data element and every single AI result needs to be reviewed one by one by one, right?
Which then it sort of degrades the general value, because how do you do that then you still have a human, what value are you adding?
But general supervision is to say like, you have to look at overall quality metrics, overall performance, you have to have measurements for outliers, like backup systems for when something goes wrong. Like that, I think is the direction that things are going.
And it's kind of similar, like Michael said, to self-driving cars, where they have these offsite operators, they can manage when something is a problem. But 95% of the time things are running pretty smoothly.
Thoughts about Strawberry o1 results
James Wang:
And this one is kind of a side thing, just because it came up recently, but I don't know if you heard about some of the Strawberry o1 results and clinical benchmarks and stuff. So yeah, I mean, in terms of response, I don't know, but a few Substack writers and other folks that got more picked up by the media and whatnot, noted that some of the benchmarks seemed cherry picked.
(As a note: the above linked articles by and are great in illustrating some of the challenges of deploying AI in medicine without great care.)
And also, the model was incredibly unstable in terms of what the actual results are.
So you do it, you run it like seven times, and, you know, maybe three times it gets the right answer. And then the other times it does something completely different.
Joshua Reicher:
Yeah. I mean, I don't know that particular one, but we see that all the time. And again, that's why there's a whole process here for robustness. It's not just that we train a thing and then we run it and we got a good result and we move on.
James Wang:
Right.
Joshua Reicher:
You run the same cases in slightly different iterations of the algorithm. You run basic non-AI algorithms as comparators. You know what a baseline performance is. You have some theoretical motivation for what within the data is actually going to drive improved results. So I think in some ways we probably oversimplify testing in the general world.
I have some friends that work in tech that work on ads and marketing and stuff like that. And if something goes wrong, there's some financial numbers that get messed up for the quarter, but it's not like killing people in the same way. So there's some greater flexibility in experimentation.
And here, you can't just train and then run the model. Like you have to really do that. That sort of full process of validation we do the same way in other regulated industries.
What is IMVARIA?
James Wang:
And maybe I should have this up front, but with your own words, do you want to describe what IMVARIA is about? Both specifically in terms of like literally, but also philosophically like what you guys are going after?
Michael Muelly:
Yeah, IMVARIA stands for image variance, right? So that variance in the interpretation of those images is really what we're trying to reduce, not just images, but data ultimately. But yeah, solving those problems where you can make a big difference with AI compared to the standard of care.
I think that's really what we're going after. That's what enables the value to allow a company to continue building those technologies.
Joshua Reicher:
In general, what we are doing is applying modern artificial intelligence technologies in serious and challenging disease areas, areas of high unmet need to help improve, empower clinicians and ultimately improve outcomes for patients.
We think that there are great opportunities in a wide range of different use cases and that the time is now for translational science to bring what has been a decade of fantastic research in this field actually to the “bedside” to apply it in the real world.
How would you talk to other clinicians about AI and medicine?
James Wang:
And maybe along that line, since you both are clinicians yourselves and many clinicians have been suspicious [of AI]—in part because a bunch of these computer scientists or whatever who have never talked to a patient are just running around and doing stuff.
How would you talk to your colleagues about AI and medicine and make it understandable to them?
Like what's the big benefit and what they should watch out for?
Michael Muelly:
I think we're trying to take that approach of education here, helping them understand what those technologies are capable of, but then also some of those limitations, right?
Because I think it's going to help them also put the tests in context and be able to place it better.
And we've found that to be pretty effective. There's a lot of interest in more education around how does AI work on a more fundamental level?
Joshua Reicher:
Yeah, I think there was so much hype in that early 2010s period and a lot of language about replacing doctors and breaking things and all that stuff.
That doesn't generally go over well with doctors.
Michael Muelly:
That does not help, no.
Joshua Reicher:
They tend to be quite conservative. And it's hard to adopt new technologies as a clinician.
You are essentially taking a risk on behalf of your patients. And so that's not something that comes easily and for very good reasons.
And so I think it's going through those formal processes of scientific development, validation, getting input from clinicians as you do development, regulatory and other sort of processes that are required, publications, peer review.
All those pieces that sound kind of slow and boring are very, very relevant here. And I can say even from our own experience, the difference between saying I've developed this cool tool versus here are our 20 abstracts and publications and an FDA authorization.
Those things count a lot and for good reason to clinicians. And so there hasn't really been a ton to date despite, I think, the headlines. And so it is still relatively new.
We get comments from a lot of clinicians of this is the first time I've actually had someone approaching me about using AI in clinical practice. And so I think there's still a lot of education to be had.
Why do you think radiology in particular has been resistant to adopting AI?
James Wang:
Radiology is an interesting one. I mean, both of you have specialties there. And it's in particular has had a lot of, I forget which journal articles, but there's been a couple in terms of editorializing about how dangerous specifically AI is.
Why do you think radiology in particular has been so resistant in terms of this?
Michael Muelly:
Most of the technologies that are out there right now just don't add a lot of value. So there's just really a handful of technologies out there. Hemorrhage detection for head CTs, stroke detection for head CTs, PE detection, lung nodules, all things that we actually do quite well as radiologists already.
And for that reason, no radiologist has really felt the need for like, oh, we need this technology. We can't work without it anymore. That's ultimately from lack of demand.
But it's really that selection of like, how do we actually solve the problems that the radiologist has as opposed to just applying the technology?
I don't know if I'm going to get myself in trouble, but I really think Vinod Khosla and Geoff Hinton getting out there and saying all radiologists are going to be out of work in five years, ten years ago, didn’t help generate positive interest from the clinical community.
Joshua Reicher:
I mean, the problem was that was really the first introduction to AI, I think, for most clinicians was seeing those headlines. And for radiologists, they were called out in particular.
So I think it was a gut check reaction of like, well, if you say that, then screw you. And this sort of pushback against it. And it takes a lot then to overcome that initial sort of interaction. I think clinicians have frustration with tech in general for a number of other reasons. And so there's a lot of skepticism.
If you look back at the history of the last 70 years or so, numerous technology companies over that time have claimed we are going to come in and solve X in medicine.
And they've pretty much all come and gone with rare exception, including a lot of companies like Intel and Pac-Bell, like a lot of companies have come and gone. So I think a lot of doctors have that intuition and don't necessarily get super excited about those hype cycles.
And I do think there's this sort of challenge that we've all had at various points of, yeah, someone who does not have any clinical knowledge literally coming in and saying like, oh yeah, I could build something that does this tomorrow.
And it's like, well, you're probably missing out on quite a bit here.
And the difference between recognizing an image of a cat versus a dog is not the same as this sort of challenging interpretation of, you know, multidimensional imaging, but also within the clinical context and knowing the demographics of the patient, like, you know, just things like age and incorporating that together into a picture of sort of statistical commonality of diseases.
And, you know, there's a lot of other factors beyond just pure pattern recognition and imaging.
And I think ultimately in today's world, radiologists are doctors. We go to medical school, you go to residency, you do take care of patients for many years and diagnostic imaging interpretation is only a part of the overall practice. And I think that's often sort of skipped over.
And I think this is a very like a side comment, but I also think that the area that's had historically the most work in the tech world has been mammography. And mammography is one of, if not the only field in radiology that is really pattern recognition.
Like everything else, there's many layers of complexity, but mammography to a large degree is you're looking for certain patterns and you've got to find those patterns and it's very high volume.
So I think people take that experience and then extend it to everything else. And it's not at all a representative experience.
James Wang:
So it sounds like you think radiologists shouldn't be too worried about their jobs at the moment.
Joshua Reicher:
I don't personally find they are. Honestly, when I talk to most radiologists, they sort of, if anything, laugh it off.
Like I don't hear a lot of fear from people in the field these days.
Michael Muelly:
Yeah. I think if anything, people would love it if AI could autonomously read some of the more routine studies at this point anyway.
So I don't think there's a fear.
I think there's just a lack of offerings on the other side.
Joshua Reicher:
Yeah. I think of a few that we do use in practice that Michael mentioned, my personal experience and I think the experience of most of my colleagues has been that they're really not that great.
Either they don't work that well, they're not super robust or they're not particularly helpful where they sit in the workflow.
So I think the result is not necessarily, I feel like we've moved past the sort of era of pushback and more now it's just like, is this actually going to help me?
Michael Muelly:
Yeah.
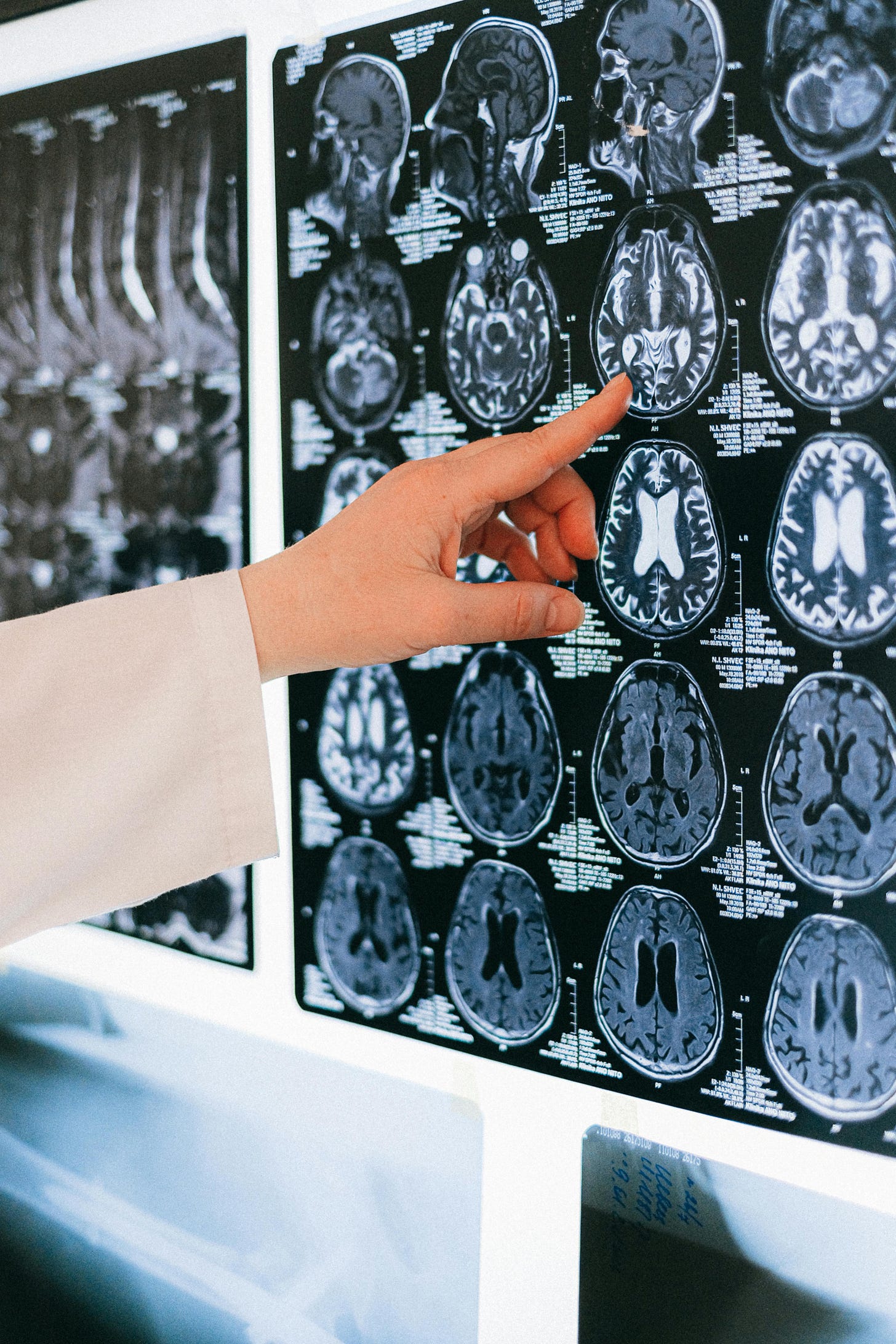
What does a radiologist actually do?
James Wang:
Describe what the radiologist's job is because I think a lot of people don't understand it. They just sort of think about it as, oh, I'm looking at an image and I write down “yes”, “no”, and then you move to the next image and then you write down “yes”, “no.”
I think that's a lot of people's interpretation of what radiologists do.
So maybe elaborate a little bit more on that.
Michael Muelly:
Yeah. I mean, a lot of times you can't just put things together based on just a single image. You need to understand the biology underlying, the anatomy, physiology and how those things would come together in the image.
Then you really have to take different data sources to ultimately make that diagnosis, which is what the radiologist does.
We provide an image-based diagnosis of the disease.
Joshua Reicher:
I think one of the questions they hammer into you throughout medical training is this question of quote, how does it change management?
That's something that I think we all have in the back of our heads at all times.
So while yes, we are looking to characterize the disease or lack thereof through imaging, ultimately we are still thinking about what can I do that's going to help make a next step here for the patient? So a lot of it comes down to, is this the right type of image? Do we need to move to a different type of imaging to help answer a question here? Does the patient need to see a different type of specialist? Is there some additional sort of recommendation that I can give to the clinician to take care of that patient that's going to move them forward?
And in many ways, the disease characterization is almost secondary. You're really looking to sort of solve the problem. And it's really relatively rare that you truly make a diagnosis based exclusively on medicalimaging.
So a lot of it is combining what is the pattern that I see? What is the reason for this study having been performed? And how does that feed forward into what is the best next step for this patient?
So even something like appendicitis, I tell people like, you don't really need even a doctor for that [diagnosis]. Like most people could probably figure out appendicitis just based on their own learning and understanding.
So you certainly don't need AI for it.
What you need your doctor, including your radiologist for is to say, are there additional complications from this? Is there a particular type of surgeon that needs to get involved? It's all of this sort of next level information.
And radiologists are kind of funny, because, while they're by percentage somewhat less patient-facing, that's also not quite an accurate characterization because most radiologists do procedures and see patients in person as well.
We are generalists because you have to know every organ system and all the different things that can go wrong. And so it's incorporating all that information to think about what is the best management step for the patient.
Michael Muelly:
And that actually goes even earlier in the chain, like just acquiring the images, right? Ultimately radiologists create those protocols.
That's not something we do every day, but the way that the images are acquired in order to be able to make the best diagnosis is also determined by radiologists.
Ultimately, we read those images that we get.
Joshua Reicher:
Yeah. I think another thing, and this is just true for medicine in general, is I think there's a, when you come into it, there's a bit of a saying that you thought your job is to diagnose disease.
Patient comes in and you're Dr. House and they have this story and then you figure it out and like, that's kind of how you think about it.
But if you just think about it, just statistically the vast majority of interactions are patients with a chronic known disorder, right? 80, 90% of interactions are patients that you know exactly what they have and what you're doing is looking for secondary complications considering changes in their treatment and management.
Michael Muelly:
Yeah.
Joshua Reicher:
The diagnosis like true, you know, primary diagnosis actually is kind of a minority of what you do, including as a radiologist.
So a lot of the work is follow up changes, you know, what other imaging recommendations, et cetera.
James Wang:
So you're saying that House is not an accurate description of what you do in terms of breaking into people's houses.
Joshua Reicher:
It's more like Scrubs. That’s the joke.
Michael Muelly:
Scrubs definitely a lot better.
What has been the problem with clinical decision tools in the past?
James Wang:
So a lot of, I mean you both talked about this too, but a lot of clinical decision tools, like [clinical decision] aides, all these things that, you know, have been thrown to doctors have all, a lot failed quite badly in the past.
So what do you think was the core problem previously and why do you think it's different now or do you think it's different now?
I assume you think it's different now given your area.
Michael Muelly:
Well it's really, you need to find the right areas, right? You need to find the area where today we cannot make a good assessment, but the AI model has the capability to help us improve that.
And the initial applications just didn't focus on the right applications. And that's really what led to, it's almost like an AI winter for radiology already.
James Wang:
Right.
Joshua Reicher:
Yeah. I think, I think there were very unrealistic expectations about what this process looks like and, you know, we work with folks who've had experience in other, you know, highly regulated high-risk types of industries like nuclear and certain financial, you know, areas, stuff like that.
And it's the same sort of issues, which is you can't just sort of train a model and send it out there.
Like there's a process and there's a reason for the process. And so I think there were a lot of unrealistic expectations about, oh, we're just going to solve all this tomorrow.
And even if, even if you technically could, it still is going to take time to run clinical studies, clinical protocols, do all that stuff. So I think there's no bypassing that. And I think we had a lot of people kind of come into the field and then get frustrated that we couldn't get this all done in 18 months.
Do you think EMR data could become a big deal within AI and healthcare?
James Wang:
Speaking of everything solved tomorrow, EMRs and EMRs in medicine and EMRs in medicine and data analysis, big data, AI, the descriptions have evolved through time.
What do you think about the idea of EMR data and that turning into something big here?
Michael Muelly:
EMR data is very, very messy. So there's the objective data in the EMR, right? You know, labs and all that.And I think there's certainly some value there. You do need that at a larger scale, but then the narratives, they typically don't add very much that you find in an EMR.
Joshua Reicher:
Yeah, I mean, both of us have done a lot of research with EHR/EMR data in the past, and I think it's, I don't want to say it's completely without value, but I think it is mostly without value. If you've ever looked at your own medical record, the amount of just inaccuracy and stuff in there is disheartening to say the least.
And I think the reality is the way that, the way that those record systems evolved and the way that you are sort of intended to record information in an EHR is almost entirely centered around our kind of billing and insurance system.
And even that, it's done poorly. So it just doesn't leave a whole lot there that I think is super meaningful or valuable in terms of data.
James Wang:
Yeah, I got a fun project while I was at Georgia Tech doing various things and yeah, it was helping UnitedHealthcare with some of this stuff. And yeah, FHIR, SNOMED, supposed standardization, meaning let's just stuff everything in and everything.
And I very quickly found that, yeah, none of the data seemed right.
All of it was contradictory and the attempt to decontradict it really didn't go anywhere.
Michael Muelly:
Yeah.
Joshua Reicher:
I mean, I've thought for years about how we almost need like a separate system for the actual like medical recording because it is helpful.
I mean, particularly as a doctor, like if someone does keep good notes, which is rare, it's very helpful then moving forward, especially as patients move around all the time and stuff.
But today's system really does not do that. And it's still mostly set around in PDF and fax machines and stuff. I mean even the electronic systems are independently electronic, but they don't communicate with each other even within one organization.
So it's electronic only in the most minimal sort of definition.
What should not be replaced in AI and medicine in the near term?
James Wang:
All right. Well, where do you think AI should not go in medicine and what do you think should not be replaced?
And I mean, we already talked a little bit about current standards, how things are going, but are there any areas that you can think of that even with advancement in technology in the next two, five, whatever years?
I won't ask ten because who knows what it will look like by then, but in the medium to near term, what do you think probably shouldn't be replaced?
Michael Muelly:
Yeah, I don't think you want to replace the human-in-the-loop somewhere, right?
Just because it takes a while for those technologies to prove themselves and to become accepted. And not just in the medical community, but ultimately in society, right? If you're asking people to trust the results that no human has reviewed. So yeah, I think humans are not going to be replaced anytime soon.
Joshua Reicher:
I have a lot of concerns about generative AI. It depends on the particular use case. You know, I don't think for front end scheduling things are sure, but we've already seen some expansion to try to do denoising and imaging, which we've worried about creating fantasy lesions and images, which is totally possible.
And you know, things like initial intake and psychotherapy, all sorts of stuff that you have a lot less control over output of these gen AI systems.
So I think we're going to need certainly some more guardrails there, or at least patient understanding and transparency of what it is they're interacting with. Because that's the type of thing that like, there's only so much control you can put around those systems.
Will OpenAI and other foundational model companies take over in medicine?
James Wang:
We've covered a lot, but let's do the “stupid questions” category.
Do you think foundational model companies like OpenAI and similar will just take over these application areas entirely with the growing power [of models] and, you know, Strawberry o1 saying that they can…
I don't want to misquote them or whatnot, but basically has an equivalent of a medical school degree?
Michael Muelly:
Well, I mean, if you have general AI, then sure, all bets are off, right? So if they do that, then yes, they will take over the world.
Do I think that we are anywhere near that point? Not really. So I don't think OpenAI, any of the large foundation model companies focusing on very specific applications and ultimately needing those examples, right? Because those are rare diseases that just don't occur very often.
So ChatGPT is not going to have a lot of data on some of these outliers. So yeah, I don't think there's any risk unless we really are talking about gen AI, but yeah, that's not going to happen anytime.
Joshua Reicher:
Yeah, nothing to add.
Why won’t China be the leaders in AI and healthcare?
James Wang:
So this has become less of a popular take, but in the past, everyone always said China, other countries, especially with less privacy and more centralized health data would be the ones who would take the lead for AI and healthcare and run off with it just because we're so restricted in the US. It's so scattered in terms of data. What do you think of that?
Michael Muelly:
I think China specifically, they have a data quality problem in most of the data generated in the healthcare system because the general standard of record keeping there is probably not quite the same.
And then really your data just becomes noise. So I don't think they have much of an advantage on the technical side just because they have more data points potentially.
Joshua Reicher:
Only other minor comment I have is that if you have anything that is regulated within the US, there's a basically statutory requirement that at least 50% of the data come from the United States.
And agencies like the FDA in general are quite skeptical of data from certain countries. So it's not just a technical question.
There's other barriers that I think will make it challenging, at least within the United States.
Impact of simulated or synthetic data in healthcare
James Wang:
I think this one might have gotten hit already, but what do you think of simulated or synthetic data and how that would affect AI and healthcare?
Michael Muelly:
Well, you're still just generating something that is in the breadth of your training domain. So you're not generating anything new, but the problem also is you cannot control what exactly you generate.
So you may generate a head CT, but there may be a lesion in there or something that simulates a lesion that could have an impact on treatment.
So I'm skeptical for those reasons.
Then the question is also the value. What's the value of the synthetic data? Not very high.
Joshua Reicher:
Yep, nothing to add.
James Wang:
Did you see that Nature paper, I think, last year where they were talking about the bigger the models got and the more instructable they are, the stupider they get for the simple problems.
Michael Muelly:
Yeah.
Joshua Reicher:
Yeah, it's going to be interesting.
Michael Muelly:
Yeah, I think scaling the models is not really feasible anymore. Computationally, the data centers are so large now. You're growing exponentially in your computer requirements. So it can't go up that much anymore.
Joshua Reicher:
When I talked to a colleague at Google the last time we got lunch a couple months ago, he's basically converted all his work. He's not actually training models anymore. Everything is fine-tuning and context switching and stuff like that.
Michael Muelly:
Yeah.
Joshua Reicher:
You wonder if that's just where things are headed. I don't know. We've got folks on our end who want to train foundational models in medical imaging. There's some reasons to consider stuff like that. But yeah, you do wonder if some of that core layer is going to end up being a little more uniform.
Final thoughts
James Wang:
I mean, it's just a lot of folks. And it's going to be a challenge in the book as well. A lot of folks’s encounter with AI and encounter with AI in healthcare has been a lot of the claims by the foundational model companies that they can do all of this just out of the box.
Or whatever fine-tune, we can do this out of the box or whatever.
And I think it's built up a lot of distrust, especially how it's actually performed. So it's been hard to differentiate from folks what you're doing. And yeah, it's deep learning, but it's not an LLM.
Michael Muelly:
It's not an LLM, exactly.
Joshua Reicher:
We've had to do, also, I think just to emphasize that, do more customization than we anticipated. Like our main machine learning engineer has had to do code commits to public Python repositories and stuff.
Michael Muelly:
Yeah, yeah.
Joshua Reicher:
They didn't have a way to deal with, you know, certain approaches to medical image training. So it clearly still requires a moderate amount of kind of customized work for a lot of these situations.
James Wang:
Yeah, so I mean, quite obviously, you can't just, again, which we addressed, be a non-subject matter expert, take a model out of the box, throw in some stuff, and hope that it comes out with something really good.
Michael Muelly:
Even if you did, right, nobody's going to accept this, right? In order for it to be accepted, you need all those extra layers around it.
Joshua Reicher:
Yeah.
James Wang:
Well, I mean, agreed, though, I think we're still actually seeing a lot of companies on our side that more or less are 80/20 doing that. Where they're just like, oh, we got this proprietary dataset and we have this thing that we tuned. It's like, how did you tune it?
It's like, well, we didn't really. We just have this thing out of the box. And it seems like it works really well within our validation set that came from the same dataset, right?
Michael Muelly:
Yeah.
Joshua Reicher:
Yes.
Michael Muelly:
Terrible. Yeah.
Michael Muelly:
You're going to have to have a ChatGPT summary at the top of this thing, I think.
James Wang:
Yeah, that would be kind of funny in terms of doing it.
I found that some of these models have gotten really, like, just like the Nature paper said, they've gotten really kind of poor at some of the normal stuff that you would kind of expect them to do. The summary for some of this stuff, in terms of output, has gotten terrible.
Michael Muelly:
Yeah.
Joshua Reicher:
I don't know what it is that they expect. So, like, when you look at OpenAI’s valuation, I was just like, I don't, I just fundamentally don't understand, like, what they think this is going to be. There are clearly some use cases. I use ChatGPT a few times a week, I would say. Maybe I'm missing it, but I haven't seen it just yet.
Michael Muelly:
It's not going to replace whole professions right at this point.
Joshua Reicher:
No.
James Wang:
Well, that’s the bet you have to make, and we’ll see. If you calculate the implied valuation, they’ll be like Salesforce in four years. This round means they’ll be Salesforce next year…
Thanks for reading!
I hope you enjoyed this interview.
Once again, this is a preview of the type of content in my book.
If you’d like to learn more about AI’s past, present, and future in an easy to understand way, follow updates on the book’s release below!
Share this post