While researching and writing my book What You Need To Know About AI, I’ve had some great conversations with experts with unique, first-hand perspectives on AI. I’ll be sharing some of these interviews here because I think some of you will enjoy reading them in full, and it’ll give a taste of what’s to come in the book.
Here, we dive into AI in finance with Alex Campbell (see his fascinating bio below). You can read the full transcript below or listen to the conversation on your preferred podcast platform.
You can also check out some of the previous interviews I’ve published:
Dr. Joshua Reicher and Dr. Michael Muelly, Co-Founders of IMVARIA, about the use of AI in clinical practice
Tomide Adesanmi, Co-Founder and CEO of CircuitMind, about the use of AI in electronic hardware design.
If you’d like to get updates on the book’s development and release, sign up below:
Alex Campbell was the head of the commodities team at Bridgewater Associates, and before that was a prop trader at Lehman Brothers in 2007. After Bridgewater, he founded Black Snow Capital, his hedge fund, and is now the Founder and CEO of Rose.ai, a data platform that helps users find, visualize, and share data, especially geared towards financial analysts and other decision makers. He also writes Campbell Ramble, a popular Substack about finance and markets.
Topics Discussed
Joining Lehman Brothers in 2007…
Experiences working at Bridgewater set the stage for creating Rose.ai
From starting a fund to starting a tech company for finance companies
Bridgewater Nerd Talk
Building Rose.ai with lived experience as a tech-savvy financier
How is the finance mindset different than the technology mindset?
Will this take away people’s jobs?
What will be the challenge for finance adopting AI?
Why not fully automate trading?
Adding productivity vs. adding or losing jobs
Is this the “Age of the Idea Guy?”
Some final thoughts against doomerism
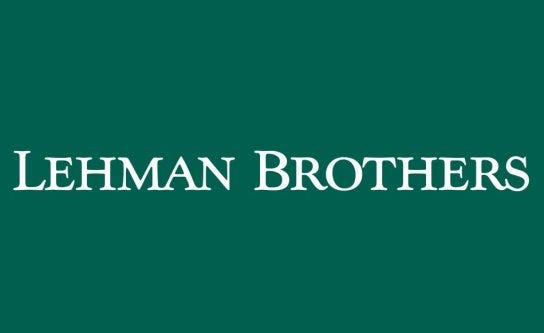
Joining Lehman Brothers in 2007…
James:
Glad to have you, Alex. I think it'd be great if you just jumped in and talked briefly about your background.
Alex:
Yeah, I got my start as a proprietary trader coming out of grad school. I was always very interested in math and game theory.
I was a failed game theorist and a very good fantasy baseball player. Given that, I joined Lehman in 2007 thinking, "Maybe they'll let me punt markets." I talked my way into the prop desk.
In three months, someone got fired, and I got his portfolio. Nine months later, I was up $10 million and the bank was dead. And so, it was a very interesting first job experience.
I was doing the kind of thing they don't let you do anymore post-Volcker. Converts, options, implied, realized, a little bit of commodities back then too. And it was really a fire hose, illustrating the notion of a financial institution as an information processing unit.
We were called the Risk Hub. Our job was to look across the bank and look across all the different crazy products that were being traded and take bespoke or prop positions. And it was very liberating and freeing, but also harrowing because you're basically day trading on some level.
And I didn't really have a good rooting in macro for my long-term views.
I would come to a view and then I would start trading it—and then I would kind of get lost. I, ironically, ended up buying some of the TIPS that I think Bridgewater was selling in 2007 and got carried out on that trade [meaning bad things happened] when they didn't revert as fast as I wanted them to. It was stupid stuff like taking the ratio of TLT to TIPS.
Experiences working at Bridgewater set the stage for creating Rose.ai
Alex:
It wasn't like anything fancy. And so when I went to Bridgewater, which is how we know each other, it was after grad school and business school. It was like catnip for me. It was a place where getting all the data was like the order of the day. And if you got the data and you understood the world and you modeled it, the trades were kind of obvious.
At least that was the notional thinking. And so I had a really good run there. Amazing place. I got tons of mentorship from the top guys. I ended up doing a project called Dots, which is kind of like AI on people. That was marginally controversial, but mostly it was just data gathering, predictions, that kind of stuff.
You know, it gave me some access probably for my time to the top guys so that when they started looking for someone to help them run the oil book and the commodities book, you know, some guy had gone into Systems and it was kind of sitting there just like ticking over. I kind of jumped at the chance.
And so now I've been there for three years and I'm not really managing $40 billion, but I'm kind of managing $40 billion. You know, James, how Bridgewater is where it's like no one's really managing it, but everyone's managing it. But you know, I'm in the room being like, "I think we should buy ourselves oil or whatever." And I just became obsessed with how hard it was to do the job in a way.
It was like being great at macro was like 2% of what we did. And like finding, cleaning, warehousing, modeling, stitching, testing, visualizing data was like the whole fricking ballgame. And we would spend enormous amounts of money on this as a core competitive advantage. And also a lot of our alpha was other people in different departments or different teams creating content that then fed into your models or whatever.
So you can imagine if you're trying to figure out what's going on in the world of oil, like what's happening with growth in China is pretty important. That wasn't my job, that was somebody else's job. I could just go pull their thoughts into my spreadsheet or my notebook or whatever.
And that's really set the tone for what I spent the last nine years on, which is Rose.ai, which is an AI company to help financial institutions find data, store it, warehouse it, clean it, and use it in systematic processes.
But in the long term, it’s also a data marketplace—a place that you can go and buy data. You don't have to buy a $50,000 license, you can buy an individual time series. If someone cleans up something that's kind of cool, they can hopefully sell it on to somebody else in that very kind of knowledge graph way that we thought about the world at the old shop.
And so I left, it was a little bit early, this was 2015, Word2Vec had just come out. And Word2Vec to me was really the beginning of the end for, “AI can't do this.”
Because if you're used to working with highly dimensional data and someone says, "Hey, if you've got enough columns, we can actually create meaning out of the difference between king and queen and man and woman. There's some sort of vector that relates those two things." To me, everything else is just downstream data munging, training, that kind of stuff.
But it was way too early to build anything functional. And so I kind of focused on running my own fund for about five years.
From starting a fund to starting a tech company for finance companies
Alex:
We tried to take a bearish view on the Chinese credit system, argued that people should buy gold, sell RMB, sell US bonds, trades that worked out very well, but I kind of didn't make it as a fund manager.
It just didn't have enough assets, restrictions, things about not competing, stuff like that, which are all fair. And I just got a real lesson in how institutionalized buy side is right now.
And you can't go skiing in Switzerland and find someone who writes you a $20 million check and you're in business, like back in the '90s. But that also then built into what we do with Rose, because we're really trying to solve that pain of, "Look, you're a great investor, you're a great trader. Why should you spend a year building data infrastructure? It makes no sense. You're probably not even good at data infrastructure. Why should you have to find some quant to help a quant to help a quant just to get data into your spreadsheet?"
So 2022 comes around, we have formalized the tool into a product, we raise some venture money, and then the AI finally kind of woke up. And so over the last couple of years, we've been really focused on using AI as an interface layer to make it joyful and easy to interact with data and find what you want and ask complex queries.
The idea is like you ask a question, get a chart, which has kind of always been the dream. It's taken a lot longer than I thought to build it, but it actually works. And so it's cool to see that.
And it's also cool to just see a lot of the stuff with AI coming that I think will be radically beneficial to the world in terms of welfare, in terms of productivity, in terms of... I mean, I use this stuff every day in my life.
And the world that's coming, I think a lot of people just don't really appreciate. But it's also great because the things that the robots do really well tend to be things that humans do poorly and vice versa.
And so I'm one of those people that sees a big complement between the human and the machine and kind of how we evolve. And so I'm pretty bullish on the future.
The things that the robots do really well tend to be things that humans do poorly and vice versa.
Bridgewater Nerd Talk
James:
Awesome. And that was a great introduction. But I'll just comment, yes, I echo your pain in trying to explain to anyone how Bridgewater's investment structure works.
It's like, what is an IA? Why is everyone an IA? Where are your portfolio managers? Are you a portfolio manager?
Alex:
Yeah, complicated.
James:
But I also echo the other point. Someone had asked me, it's like, yeah, is this all just X? Is this all just momentum? Is this all just—whatever? Is this all just whatever for what Bridgewater did?
And usually my answer to them, which goes towards your point with Rose.ai, is no, it's just stuff that's a pain in the ass to do.
Did you know that this BLS metric changed its methodology from this year to this year and no one actually, whatever it is, and do you know that the revisions weren't specifically posted on this? There's a lot of stuff that is just blood, sweat, and tears.
That's the alpha generation and not anything magical or cheating or whatever it is now that some of these random books have come out. So yeah, I think that echoes exactly what you’re saying in terms of the specific area that you're trying to jump into and what you're trying to do.
Building Rose.ai with lived experience as a tech-savvy financier
James:
But I guess there's a couple of questions that go off of that. This is not the first initiative that, in general, has been done and that I've seen in terms of random PhDs basically saying, “Hey, I'm going to bring technology to finance.”
And yeah, that's a new concept. I don't think anyone's ever heard of it before. I think it's a great idea. Will you fund us?
So what makes this approach different and what part of your background sort of informed how you're approaching Rose.ai?
Alex:
Yeah, I think a lot of people run the same route in trying to kind of end around the hard work that you kind of mentioned. I call it pain alpha.
So very similar mindset in a sense. And the framework that I use is California versus New York.
So I went to school out of California. I got that entrepreneur bug. I have a bunch of friends, Burning Man, yada, yada.
That philosophy is great at building massive internet-scale technology first companies that light money on fire for five years and then make tons of money down the line.
And there's a through line for that and a narrative for that and really a cookie cutter kind of approach in a sense when you think about, you see these companies that go ABCDE… IPO… and then $500mm+ company over like 10 years.
Most of those companies that try to do finance fall victim to hubris in a couple of ways.
One, they either try to fight Bloomberg head on, which doesn't make any sense. Bloomberg is an institution. It's a utility. It's a source of truth for a lot of these financial institutions.
You should try to make love to Bloomberg, not try to kill it.
Same thing with spreadsheets.
So there's this huge animosity against Excel, against spreadsheets, which is the dumbest thing I've ever heard because finance got started when the Medicis did double-entry bookkeeping, right?
And running around and that kind of thing. I've actually seen the ledger for JP Morgan. If you go to the Morgan library, you can pull the records. You can find the Knickerbocker Trust Bailout napkin that he made everybody sign, the piece of paper.
You can find the first ledger from when Morgan rolled the banking over from another partnership to what became JP Morgan. And it's like, you know, four partners have a piece of paper. One of them put some like land on it from 1666 or on it or whatever. And on the second page, it's like Mr. Smith bought some shares on account, basically trading the balance sheet like a fricking hedge fund, right?
And so I think that there's this real cultural deep, you know, kind of learning that most people in finance get hazed into in their first year as a banker or a consultant or a trader or whatever it is, which is like, you do not fuck with the money.
You do not fuck with the returns. You do not fuck with the outputs.
They either try to fight Bloomberg head on, which doesn't make any sense. Bloomberg is an institution. It's a utility. It's a source of truth for a lot of these financial institutions.
You should try to make love to Bloomberg, not try to kill it.
You know, everything we do is kind of open book at some level because a counterparty or a regulator or an investor or whoever will sue you or whatever. And you have to show them all your work.
This happened to me when I clicked the wrong button on my Form ADV when I was doing my tiny little hedge fund. And I get a call from the SEC three years later being like, are you marketing to retail punters and blah, blah, blah, blah.
I'm like, no, no, here's all the accredited investors. Here's all our reports. You know, we got through it, but it's like a good example of this weird world you enter when you professionally manage other people's money or you're the bank.
And the irony is, you know, you see Saylor out there running around selling, you know, realized versus implied vol arm to everyone who's investing in an $MSTR as if that's not a hedge fund, right? But okay, fine.
I'm going to back away from that for a second and say that, you know, that's why you have to use spreadsheets in a way.
That's why you have to see rather than tell trade reconciliation, account management, audit, compliance, all these things are naturally lists. And so Excel's not going anywhere.
And so if you just start with those two basic presumptions, right? One is that like Excel's not going anywhere to like Bloomberg's not going anywhere.
And three, like the work that's being done by all of these idiots or whatever to go clean up this data from Bloomberg and Excel is actually valuable.
It really changes how you approach the whole thing because I came to it from a practitioner's lens like I'm a coder, but I'm also a spreadsheet jockey.
I'm a fundamentalist, but I also build systems. I've never kind of fit in anyone's box. And most of these technologists are like, “We think you should do your work this way. You know, like you should use our structure. You should kind of move how you work out of this environment.”
One, little do they know how hard it is to even support that environment. And then two, they try to push SaaS, which is like the worst possible business model for financial services.
Because if you know how hard it is to get one person using your software at a JPMorgan or Goldman Sachs or Bridgewater or an AQR, you do not want to do that again for every stupid team or every stupid user. Like it makes no sense.
Meanwhile, I've had clients where I've had to install Looker dashboards and Tableau dashboards. And if I want someone on the other side of the building to look at that chart, I have to get them to fill out a form and pay those guys money. It just doesn't make any sense.
Like what you have in finance is: you take a screenshot, you send it to the person. And then, if they want the file, they get the file. And so that's kind of when you combine that lack of empathy is what I usually call it with a little bit of a blindness to why all these AI companies hallucinate and don't have memory and aren't good with structured data.
You kind of end up with this kind of hole (which is a Rose-shaped hole) which is a thing that can talk to vendors, a thing that can talk to Excel, a thing that can act as a database that you want to use, that you want to talk to, but that can record when humans clean up or model or organize data.
It sounds like not the biggest deal in the world, but you sort of do the math of: you go into one of these data vendors and ask what's inflation… And they have more kinds of inflation than there are countries on earth.
You know, you have the World Bank. Or rural, urban, ex-energy, ex-food. And PPI or GDP deflators… What's inflation? Nobody knows.
And answering that one question one time might take you 20 minutes, and you don't think about it again. Now do it for every country on earth, it starts to become a little more daunting.
Now do all the kinds of inflation for every country on earth, it gets really daunting. Now think about how we do that every month over and over and over again, and you go, "Whoa, I have no time to do anything else."
And that's, if I took anything from Bridgewater, it was just that secret of compounding, which is like, no, you get to the end of that process, you get to the end of that loop, encode that information, record it, store it, put it somewhere where someone can access it, and then lever on top of it so you don't spend that 20 minutes over and over and over again.
I got very intolerant of that. And so that's our approach. It means that some of these California people don't like us, because I go to these meetings and I'm like, "We do B2B SaaS." And I'm like, "Well, we don't do SaaS."
And they're like, uh…
And it means that some of the clients that we work with, who are very big, fancy financial institutions, have a guy or a gal who's trying to build their own version of the warehouse that does all the things, and so their go-to-market kind of sucks.
But as the world has evolved, I mean, oh my God, when I left Bridgewater in 2015 versus today, people used to throw phones at me, they would laugh at me, just crazy shit from me, just even talking about this idea.
And now they're like, "Oh, it's so cool. Are you raising $200 million or whatever?" And I'm like, "No, no, no, that's Ilya. He's training some matrices." But I love it. I think the world has kind of validated the thesis.
Building a great business on top of it is kind of the point that we're at now, but that's how we do it differently. It's really trying to be symbiosis, machine plus human as opposed to human versus machine.
How is the finance mindset different than the technology mindset?
James:
Yeah, totally. And I'm not going to say who, but during my time at Berkeley and my MBA, I took a bunch of PhD seminars in computer science.
People found out, "Hey, this is what your background was." And I had a few people—one in particular—pull me aside and say, "Hey, I'm working on this thing that is meant to bring machine learning to finance." And what you’re saying perfectly resonates, which was he was really driving at, "Hey, here's how it works," et cetera.
And it was like a Spyder or RStudio kind of interface, but with drag-n-drop blocks so that these stupid finance people could use it or whatever. And I was like, "I get it, but can you make it into an Excel plugin or be able to show at least a visual spreadsheet?"
And the person's looking at me like, "You're completely insane. Why would you do that?"
I was like, "No, you don't understand. I don't want to explain how many ridiculous, crazy things we built in Excel at one point or another that Excel was never meant for, but finance works on Excel. It does not work on this thing."
Even for me, let's be clear. I'm in your class—like literally in your seminar. I know how to program. I know how to do these things, but I'm just saying I don't think you understand the finance culture.
And speaking of which, maybe you can address it more explicitly too, just so people can understand… Because I think a lot of listeners and readers and stuff are from either Silicon Valley or technology.
Maybe describe a little bit what that finance mindset is, because it does seem to be very different and tech's place, not in all hedge funds, but in most hedge funds, is quite different than what it is in, say, a startup in Silicon Valley.
I was like, "No, you don't understand. I don't want to explain how many ridiculous, crazy things we built in Excel at one point or another that Excel was never meant for, but finance works on Excel. It does not work on this thing."
Alex:
Yeah, that's a great question. I think the biggest distinction is where does the ambiguity lie and who's responsible for it?
When you're in a startup or a tech company, the answer is always yes. The answer is always, "I can do that." The answer is always, "I'll stay up. I'll figure it out. We'll get 80% of it done."
That's the opposite of how it works in finance. The reason that banking culture and trading culture or Bridgewater or whatever is so intense is that when you're managing someone else's money, even if it's just a derivative or a banking transaction, saying that you know the answer when you don't is the worst possible thing you can do.
To the extent that, at some point it becomes fraud.
You get these Archegos or whatever saying, "Oh, we have more money than we think we do." It really cuts to the core of the thing—which is you do not say things that are not true ever.
If you do, you get viciously punished.
Most people who work in finance have visceral lived experiences of trying to do the tech thing in the finance background and someone just tearing them anew. I know for me; it would be like... at one point we were trying to find EM sovereign debt crises or whatever.
I found 10 of them. I was like, "Oh, these are pretty good. I think this is 1984. This one's 1990.” Whatever.
One of our colleagues, since it was going up to the Big Man [(Ray Dalio)], he looks at this and he goes, "This is crap." I'm like, "What do you mean it's crap?" He's like, "Well, you're missing five and this one was 1972, not '73," and so on.
He had just picked up how I was operating at an 80% level and the answer had to be 100%. The expectation is 100%.
That's why people are up all night and the red lines are crazy, and you have these crazy hours. It's not that the work is that hard a lot of the time. It's the stamina required to stay at that level and guarantee outcomes and then clearly communicate what's an assumption, or what's not, or how it drives the outcome, is in all finance.
You go into banking, you go into private equity, their assumptions are about five years forward, even to a margin growth or whatever or a subscriber growth churn. They get down into the details so they can say, "Here's the thing I don't really know and everything else I'm trying to control for and give you a story for."
With Macro, I think it's even more intense because when you're dealing with a stock, you have the thing where they actually create the earnings and then you have all people punting on the earnings.
A lot of stuff in Macro, you don't even have the earnings part. You just have people punting it like currencies or whatever.
Yeah, there's a yield and you can bet against forwards, but you're just playing the blob. It's super easy to convince yourself that you know what you don't know. Just look at FinTwit.
Look at how many people are on there being like, "Oh, here's the stock that's going to go up a million percent," or, "I was right about the stock market crashing."
There's something deeply humbling about how many times when you're trading markets that you do the dumb thing and you go, "Oh my God, I did it again. I should have been more careful."
I think that that's also the opportunity because I think we have 20 years, probably a decade of people who haven't moved past Excel 2007 more or less. Now they have all these great tools to do unstructured data.
There's lots of startups doing like, "We read your PDFs and we tell you what's online," or whatever, but that's not really the problem. The problem is this weird nexus of workflow where you go and you suck data in from a PDF or from a website or from a database and then you do something to it.
You combine it with something else. You create a chart that is the synthesis of the bet or whatever and then that goes in the PowerPoint which goes in the Word file which gets in front of 5,000 people or whatever.
That notion of, "Hey, if I want to update that chart, what do I have to do?" Again, compounding.
Most shops, especially if you're just a non-systematic shop, will be like, "Well, we have an analyst and once every three months or six months or two years, he updates the numbers and runs the model."
If you're a systematic investor, that must happen every day. It must be right. When the data vendor screws up or the schema changes or the API endpoint dies, you need someone who's smart, trained, versed enough in these concepts to go and rip it apart and understand how it's built and go tell you, "Should you go sell 100 million of bonds or whatever?"
It's just a very different bar, a very different culture, a very different outcome than you're working at some tech company and maybe you spend $100 million on Facebook ads a year, so who cares about $90 million versus $100 million?
You make these decisions, and you start to see the returns. Slowly.
In finance, you can always put on enough money to blow you up in a day if you're dumb enough. You must be respectful of the obligation to your investors, the obligation to your counterparties, and then the obligation to yourself.
That's I think the culture.
If you've seen the show Industry, it reminds me of when I was working at Lehman a little bit. It's all condensed and jammed up but that notion of like, "I did a trade break. Oh my God, what's going to happen?" You know what I mean?
This client is going to leave forever and we're going to lose two million of revenue or whatever. It's just very raw and it connects money to emotions in a way that you have to have guardrails around, in my opinion.
[Finance is] just a very different bar, a very different culture, a very different outcome than you're working at some tech company and maybe you spend $100 million on Facebook ads a year, so who cares about $90 million versus $100 million?
In finance, you can always put on enough money to blow you up in a day if you're dumb enough. You must be respectful of the obligation to your investors, the obligation to your counterparties, and then the obligation to yourself.
James:
Yeah, totally. I think one of those things there that it's interesting because the thing that you're talking about is actually in other places too.
Tech has had kind of an interesting time especially with AI colliding with a lot of different industries. One of the other interviews here is going to be one with radiologists and talking about AI in medicine and whatnot.
It's the same thing that someone else had mentioned before as well where it's like self-driving cars.
It's a very different thing to screw up slightly and just whatever, put the wrong Facebook ad for a day or something like that or serve the wrong one or blah, blah, blah.
It's quite another to have a mistake in a car driving or a medical decision or, yes, like you're saying, a $100mm bond trade and having that go bad.
Very different culture. The point of it is not we have the fanciest technology and it's the most newfangled thing in the world. The point is no, it works correctly and we know that.
Alex:
Exactly.
Will this take away people’s jobs?
James:
I guess one of the questions there and it's always one of the fears for AI with all of these things.
The thing that you're describing with Rose.ai—I played around with it. It's like you have an interface, you're able to type something in and it pulls up a chart for you and everything.
The thing that reminds me of is the junior analysts and whatever that I've worked with at Bridgewater or other places. It's like, okay, that kind of can serve as that.
But I guess from your perspective, does that change things in terms of do you need fewer people? Is this going to take people's jobs away? How are you thinking about that and where do you see this going?
Alex:
Yeah, it's funny. So, we pitched when we first did our like go to market with the venture folks back in 2021, I remember pitching Sequoia and being like, look, global finance GDP is $7 trillion.
About one in seven of these people are doing this job. Leaking data, munging data, warehousing it, whatever you want to call it.
Our TAM was a trillion, right? And they were like, ah, we don't believe this.
And then I think this June they published that the TAM for AI is $10T because all these people are going to get automated. So maybe they listened a little more than I thought they did!
But I think it's a hard question because I'm a big complementarity person, not a substitution person.
We have this phrase called, “Don't trust machines,” which leads you to reflect on when the machine breaks.
So the machine breaks and you go, oh, wait, don't trust machines. You should always have a valve out.
And so I think with the radiologists and a lot of these things, car driving is interesting because you're fully automating the driver. I think with radiologists, hopefully you'll just have a bunch of robots then sending corner cases to radiologists.
But I think when people look back on this era, they will be shocked at how many bullshit processes there were. Like speaking of radiologists, I hurt a disc in my neck. I went to the guy. He told me to get a radiologist.
It took me three weeks to get the thing. I go through the thing. I get this really uncomfortable experience. I go back to the neck guy two weeks later and he's like, oh, the radiologist forgot to fill out the bloody form.
What the—you know what I mean?
Like why did I even spend all this time, like a month of my life, waiting for this radiologist report that I still don't know what it says?
I get excited about this in education and health care and the kinds of industries that are very service-oriented. Where we see productivity gains… and then they still suck.
I think we should definitely automate the heck out of those things. Like automate doctors, automate all this stuff, but with guardrails. To your point.
But I think you were saying about my business, like I think of it as right now you generally have a coder and Excel jockey in most of these institutions.
You get pure quant funds where they don't have any Excel people even though they're all in trading, account management or account regulation or whatever. You get these fundamentalist shops that don't want to code. I think you'll get kind of one job where there was two jobs.
And it used to be you were a coder or you were an analyst, right? You bought the data or you used the data. I think you'll see a merging of those two because you don't need to get intermediated by a tech person if you're a spreadsheet person.
But you can then use code. And then conversely, a lot of these tech people have a hard time making charts because they're not trained in charts, they're not visual necessarily.
They tend to be more text-based, more logical. And so our argument, while I think you'll see some automation of the extra labor that doesn't come on board, is that you won't see that much more firing actually.
What you'll see is more productivity. And then teams where you get more of a hybrid approach where the data person is also the analyst, the analyst is also the data person. And that if you can close that loop, you unlock a lot of productivity.
Because anyone who works at an asset management firm where they have a tech team who does analysis knows the pain very deeply of like, you put the JIRA ticket in, the person does the dashboard, it's wrong, you ask them to fix it, they're like, maybe they work abroad so they don't even get your email until the next day. And then it takes you three weeks to get a stupid chart out.
I think that we can just collapse that into one loop.
And if you actually start to think about buying intellectual property, which is our kind of ultimate long-term goal, it gets radically more productive. Now, it doesn't mean that you have to automate your whole team. You probably want to cut the extra fluff.
But it means that you get differentiation. You get out of this pin factory. There's someone out there who's trading milk or butter or whatever, and they're going to clean up the butter futures curve. [James editorial: if you’re not in finance, don’t worry about it—too many layers for a single link to bring erudition…]
And you don't want to do that, man. It's a pain in the butt. It's a waste of time. Like, pay that person $100. They get some income for cleaning that data. You get a clean futures curve.
Same thing with price to earnings or price to sales. You go to Yahoo and you look for price to earnings, price to sales or whatever. There's a methodology they're doing to calculate sales, a methodology to calculate earnings.
And you start poking on that and you realize how many different ways there are to calculate earnings, right? Am I doing backward-looking? Am I doing forward-looking? Am I smoothing out pro forma stuff? Yada, yada, yada.
And again, that's why the people who work at the Vikings or the CO2s of the world get paid so much, because they know how to navigate those issues in a way where they still kind of understand what's happening.
And so that's the productivity that I kind of want to see.
It's different than the Waymo productivity. The Waymo productivity is like, you push this button, you do it a certain way, the button is the steering wheel or whatever. We're going to get rid of that entirely.
It's more the unlocking of new kinds of economic activity that come when AI has solved search, or AI has solved [data visualization], or AI has solved warehousing. And so, if you do all of those three things, you just unleash this new form of buying and selling knowledge.
James:
Yeah, totally. And I think what I'm hearing from you too is it's both people becoming more generalist and also someone still needs to be responsible, right? Because just echoing what you're saying, it's like all of this, you know, all these different earnings methods or whatever.
It's like, can you just throw that over to an AI and hope that it comes back with the right one for the right occasion and think about it in the right way before presenting it to you?
Alex:
Right. You can get a ton of leverage from these models, but they still make mistakes all the time and they have no memory. And so, you know, like one of the best use cases I found for these AIs is like make a big list.
You just tell it like, hey, I want a big list of every this, every that. It loves doing that.
And you know, 10% will be wrong, but like it's way better than me sitting down and writing that list. And so when you start to add that to the make a bunch of code or the, you know, that kind of thing, you can see the creative aspect of it, but the reliability still isn't there.
There's no memory, there's no warehousing, there's no consistent version of truth across the different charts. You know, they change the models every five minutes and so you don't really know what you're getting. You get different outputs.
What will be the challenge for finance adopting AI?
James:
Yeah, totally. And I mean, I think one of the questions then as well is like, what do you think is the big challenge for AI being adopted within finance just more generally? From your experience—remembering both the culture of it and some of these challenges that we've talked about. Do you think it's going to be something that people trust and bring on and how will that process work?
Alex:
Yeah, I think you have a nice setup for this question…
Forget my company for just a second. It's taking a long time for the same reasons that my go-to-market is a pain in the ass. Which is you try installing anything on a computer at Barclays.
It's just not going to happen. It's just not going to work.
But you have the scaling catching up with this, the kind of penetration. And so I think, you know, in two years, let's say, right, you're going to have these models still hallucinate, but way, way less.
That won’t solve the problem of the real-time nature of markets. During our conversation, the price of Google stock just changed or Tesla—there's no perfect knowledge. You’re chasing a market that's always trading and there's different exchanges and what is the price and all that stuff that will never get solved 100%.
But you will get the thing where: if you ask it for 2024 earnings, it does an okay job. And that will probably take as long as it takes to get internally at the [big] funds.
The thing that you, that you kind of hit immediately though, is that most of these funds or banks or financial institutions cannot exfiltrate data to third party services without heavy guardrails.
And so, you know, you saw this with Zoom, you see it with Teams and Slack, these kinds of tools [running into data issues], which are even more obvious than AI, in a sense.
And, you know, it took COVID to get Zoom on everyone's computer. And then they realized, wait a second, like, why are we using Zoom and sending all the data abroad? Like, that seems kind of weird, but like, nobody cares. It's too late. It's already locked in.
I use Google Meet and people are like, “Oh, are you worried about Google having the data?” And I'm like, what are you talking about? Like kind of, but like, you know, what's the alternative? Same thing with Slack and Teams, right?
That data is going somewhere. Is it a local server? Is it a foreign server, that kind of thing? I think I don't know exactly what it is, but we need some sort of loosening or, you know, deregulation.
Again, I'm not an expert here to make it a little bit less brittle. And I think you will see a lot more implementation.
I think a lot of people are kind of selling snake oil right now, where they're kind of like wrapping very good models and creating some kind of quasi-financial bespoke products.
And then they're just levering on the fact that they can do the sales cycle, whereas Anthropic or GPT might be a little slower [to enter that market]. I don't view, “Hey, I read a bunch of PDFs and told you what's on page five,” as a competitive moat, right?
My moat is like, we cleaned up a bunch of data that the robots can't clean up. And like, we can combine it in ways that people can't. So I think you'll definitely see it being used more.
I think the question is, where are the norms? I have a Substack where I will put data in there that I'm getting from the robots. Like I wrote this thing on silver, and you know, okay, solar power, if solar power grows at 30%, like we're going to eat up all the silver on earth, but you know, it's very dependent on how much silver you need per watt. Okay.
And you know, I had one instance where the robot overestimated it by like five times. And so, my numbers were off by five times. And then, you know, somebody else came back and told me I was an idiot.
And then it's like, is it 20? And then people say it's actually 10 milligrams. And then someone says, wait a sec, the new technology uses a bunch more. It could go back to 65 or whatever.
And this is the kind of thing that you shouldn't really trust the robots on.
What you can trust robots on is, hey, I've had these assumptions that now I have vetted and I want you to think in this way and model it in this way, you know, take growth to 10 years forward and model it this way. It's done. And that is magical. That is amazing.
The like, the notion of, you know, you bring your data, you bring your logic and it just finishes the thing for you. Or someone who's not techie being like, how do I build a portfolio optimizer? And like the robot knows how to do it. And it gives you a Jupyter notebook. You can kind of run and figure out like, these things are magical.
But you know, anyone who's tried to install Python knows how unbelievably painful setting up environments is in Python. They haven't solved that problem either. So I think the consistent pain of working with technology will still be there. But a lot of the things that are really shitty and really painful will get automated away hopefully.
Why not fully automate trading?
James:
Yeah, totally. And I think that speaks towards… well, I'll ask the question directly.
It's like Rose.ai, in a way, is a very modest type of approach. Because you can argue, “Hey, maybe you should just have AI take over all of your trading and just go and implement all of this or whatever.”
And you could come out with some sort of product that helps do that. What you're doing is you're automating the really painful parts, but a human being is still going to be there, monitoring, looking at it, utilizing it, overseeing it.
I guess what I mean, again, you talked some towards it, but just explicitly, it's like, why not? Like, why don't you? Why don't we see more of these AI funds that run off and just entirely work that way?
Alex:
Yeah, I think, you know, first of all, you have to give credit to the smart quants that are out there.
So you go to AQR, you go to Jane Street, you go to Two Sigma. These are folks who have been using machine learning to create markets for 20 years, right? Renaissance, that kind of thing.
So they're not dumb. You know what I mean? They're pretty smart. And so, when you think about being good at that game, you don't just have to be better than the average person on Twitter, you have to be better than those guys. And those guys also have the tools that you have. So why aren't they better than you is one thing.
Two, I think that most people fail at the Rose level. They don't do the warehousing, they don't do the integration, and they don't do business logic in a way where the robot can drive it.
I think a lot of people have been coming to me, especially recently, as expectations go up and up and up saying, hey, can you guys train a robot to trade markets?
And it's like, of course, we can.
Like, if you were to do that, you would need our data. That's kind of why we started at the infrastructure layer. Because when I left, it wasn't like, oh, I'm going to build an AI to trade markets. Like, everyone has that idea. It's not a hard idea. It's, “Wait a second, like, Bridgewater is one of the best financial institutions on the freaking planet. And this is their hardest problem.”
And they've solved it with a lot of business processes and a lot of really smart people and, you know, having a certain way of doing things for 20 years.
But is there a way to kind of capture the methodology of like, just systemization, write it down, you know, be consistent, try to build the same models? And then AI is easy on top of that because you solve what the AI is bad at, which is hallucination.
You solved, you know, UUID reconciliation, you've solved like, as I said, these are the elements that I want, go run it in this way. And so I think at some point, we will do more trading systems, we will do more kind of bespoke analysis, you know, eventually, AGI for trading is kind of potentially on the roadmap.
When I left, it wasn't like, oh, I'm going to build an AI to trade markets. Like, everyone has that idea. It's not a hard idea. It's, “Wait a second, like, Bridgewater is one of the best financial institutions on the freaking planet. And this is their hardest problem.”
But I've always been like a big proponent of kind of how Ray Dalio thinks and does things, which is like, don't start with the hardest version of your thing, because it's going to frickin break. Just do it yourself and then try to automate the crappy parts.
And so, data integration, warehousing, custom business logic, visualization, you know, ETL, revisions, sharing, all these things, which are just incredibly nasty problems, we have solved.
And so, you know, there is a chance someday that maybe if they go to market on the like, data warehouse marketplace is so bad, we'll just be like, hey, guys, it's you know what, it's just a robot that trades markets for you, or, you know, analyze markets for you, but I don't think we're there yet.
I think it's maybe a year or two away before the robots are that smart. And you have to think about context.
Most of my experiments right now are just around getting a robot to talk like me or somebody else. So I think if you can kind of map that brain to a robot, and then you feed it rows, I think you will get the thing you're describing, which is, hey, I have a philosophy and I have data and I want to implement it in this way.
I think that there are a lot of people masquerading as, “Oh, I trained a really good model on my tweets. And now it's trading crypto!” You see this out in the world, right? And they're getting faddish, and they're getting pumped up and stuff, but they're not making money from alpha, right?
They're making money from pumping coins or monetizing their social media presence, look at Hawk Tua, that kind of thing. You know, she cleared out all her early investors immediately.
So like, it's a really, really, really hard game. It's one of the hardest games in the world. You're playing poker with the best poker players on earth in the highest stakes game in the world. And so I have a lot of respect for that game.
And so, you know, I think people will try it, but I think they will need to basically either use rows or copy rows to do it. Because you cannot manage money systematically without a warehouse. It's just impossible.
Like, you know, you imagine going to Two Sigma and being like, hey, guys, what would happen if you shut off all the SQL and all the OCaml or whatever, right? It's just like, that’s no fun.
So I think that's where we're maybe a little too ahead of people because they see the pie[-in-the-sky] and they get their eyes kind of go googly-eyed and they go, “Oh, my God, can't you do everything?”
And I'm like, no, no, I'm trying to help you like, get data into your spreadsheet. And like, oh, that seems like a really shitty thing to work on. Like, why would anybody care about that? I'm like, no, you don't understand.
It's like a really big problem. And you need to do it to do the AI for finance thing.
But I love that. Because as long as you're not too far off the path, if you do have a real pain point, and you solve it in a real way—eventually, they'll come to you, you have to stay alive for that long, and you have to fight the entrepreneurial journey and whatever. But, you know, eventually, if you have it, I think they'll come.
But I've always been like a big proponent of kind of how Ray Dalio thinks and does things, which is like, don't start with the hardest version of your thing, because it's going to frickin break. Just do it yourself and then try to automate the crappy parts.
James:
Yeah, totally. And I think just to echo some of those points, too, for some of those firms that you talked about… I've talked to some of the folks who work there, right?
I think a lot of people think you just throw things into it. And then magically, the system trades, and things happen. Profit. It's like Bridgewater being systematic. It's like, “Oh, that means that super high-tech robots are doing everything at your firm, all the time, right?”
It’s like, yeah, no. That’s not the case for any of these firms.
Even if you are trading with machine learning, someone is overseeing it and someone is being very careful about what the thing does. Because yeah, the last thing that you want is this thing to run off and do crazy things on its own, because it's spiraling out of control or whatever it is.
It’s still very much a human process. And I think that's basically what you're saying. It's like, yeah, maybe one day, all bets are off if we get AGI. Sure. But until we get to that point, human supervision is still needed.
So what you're talking about in terms of being modest… is perhaps less being modest. It's just attacking the actual problem as it stands, versus trying to do something that doesn't exist and can't work.
And like, it's just hopeful thinking, especially from folks from outside the industry.
Alex:
Yeah, because you know that OpenAI and Anthropic have a bigger training budget than you, right? And so, if it's possible to train an ASI [(Artificial Superintelligence)] to the point where it's better than anyone who's ever traded markets, blah, blah, blah, even if that works conceptually, they have to buy data.
You know what I mean? They're going to have a Bloomberg license. They're going to try to get macro data from like MacroBond or Haver or whatever. They're going to try to pull data from FRED.
They're going to need a warehouse. They're going to need to do reporting. You know what I mean? It's like, even if you automated everyone at Bridgewater into a robot, it would still use a database.
And so that's kind of where I think about myself and us as like selling coal during the Industrial Revolution. It's like, we're trying to sell hotcakes, clean data, good data. Here's some cool data for you. It doesn't matter if you're a robot or if you're a human. And if you are a robot, great.
Maybe you then monetize by selling some of your outputs into that network because you need to get capital. You know, who cares where the data is coming from if it's good data?
You know, and I think the irony is like, if you had told people 20 years ago that the best weather team on earth was at Citadel in Chicago, they'd be like, what? You know what I mean? Like, how is that the case?
Okay, well, we had this thing called the Ukraine conflict and everyone was really worried about European natural gas… and they figured out that they’d have good enough weather not to need to worry about that. And they made $10 billion on that trade!
So, it's a really hard thing for, I think, especially venture people to kind of grok because it's like, wait, you're telling me that like one weather system is worth $10 billion. And you're like, yeah, I mean, you must be Citadel to capture that $10 billion. But yes, you know what I mean?
Like markets are such that if you have a differentiated view on truth and you're early, you can make unlimited money. Well, not truly unlimited, but you know what I mean. You can make a lot. And that information has value.
And right now, we're so used to that getting commoditized or monetized within each firm. The ability for that to happen across firms and humans is—to me—something that seems obvious but will take some time for people to normalize.
James:
Totally. And I think part of the point that I'm making too is just that for that Citadel trade… someone had to have asked the question, the correct question: “Well, what about the weather in Europe this winter?”
It's like, yeah, the AI doesn't do that magically for you. It's not like you can run off and just tell it like, give me the right trade.
Alex:
Exactly. If you ask it what to do, it's like, oh, you should buy some tech stocks. It's like, okay, thanks. Basically just like every retail investor.
Adding productivity vs. adding or losing jobs
James:
Yeah, totally. I think that's right.
But at least at this point, someone still needs to come up with a trading idea. Even for finance, it seems like sometimes you just can't get the people that you need or want economically.
Recruiting is a hard thing.
And what this stuff is doing is… it's not taking away someone's job that already exists and mass automating farms of data analysts or something like that. It's just we don't have them, right?
Alex:
For sure. And also it unlocks the ability for people who live in places with way less wages to do that work. You know what I mean?
It's like, you take the first-year Bridgewater analyst job, and a lot of it's just training so you can be a higher-order thinker. But you know, a lot of that work could be done by someone who's not a fancy investment associate.
You know what I mean? It's just, are there the pipes? Is there context? Is there a sharing, you know, way of doing that?
And if you think about AI is, you know, you give it a problem, and it figures out how to break it into pieces, and then has humans do the pieces that it can't versus the pieces that it can… It's like, well, are we firing people if we're hiring, you know, four people in India versus one person in Connecticut?
Like, I don't know, we're just being more efficient on how we unlock that productivity. So, you know, I'm a big, big productivity boom kind of guy. I don't know how it'll be resolved in GDP and asset prices; it's a lot harder of a question.
So, I used to work at a photo lab, fun story. But I was like, one of the guys, you come in with your one-hour photo, and the machine would be running late. And I'd be like, “Oh, it's going to be three hours,” and people would be like yelling at me, like, “Ahh, you guys say one hour on the thing, you know,”…
It was 20 bucks for a roll of film or whatever, and then we try to sell them some cameras on the side, oh, you know, the new Olympus, I make four bucks if you buy the Olympus from me, right?
And now, I'm like, I have a camera on my phone that's better than anything I could have bought in 2000, I can send those photos anywhere in the world, I can post it on the internet. And that stuff's all basically free, right?
How much productivity has given us is really hard to measure. And I think it’s really confusing to think about. But it’s really obvious that we benefit from it in retrospect when we think about it.
How much more productivity has that given us in ways that are hard to count is really confusing, I think, to think about, but obvious in retrospect when you think about it.
Is this the “Age of the Idea Guy?”
James:
Okay, so maybe this is a bit of a challenging question where no one has the answer. But I wonder if you are going to get four analysts in India, or just one super talented analyst in Westport with these tools.
A lot of low-level stuff tends to be the thing that gets automated.
Towards that, and I don’t have the answer to this either… What happens to junior folks who used to do the grunt work? Is there still a training path, or do you end up being so automated that you can just have Ray, or whoever, with these productivity tools expanding and filling the space himself?
Alex:
I mean, you get some winnowing, right? You need less labor. I made this kind of jokey tweet the other day, “It's the age of the idea guy.”
I think it’s really, really the case. That’s part of productivity.
You see that the time to create a startup has gone from a year to a quarter to a day. Some people can create little businesses off these GPTs that print $20K a month of MRR (monthly recurring revenue) or whatever in an afternoon, and you imagine opening that up to everyone who doesn't have formal education or doesn't think in code or whatever, it's just this massive unlock.
So, I think you'll get more of almost a weird return to classical education, like more people reading books and talking as opposed to writing homework.
Homework is so stupid to me; it never made a lot of sense. Homework is basically dead, anyone who pretends it's not is kind of lying to you.
I went and talked to one of my professors from Stanford and I was like, what's your policy on AI? And he's like, we don't have a policy, we can't control it, we can't audit it, do whatever the hell you want, we have to figure out how to work around it.
And that's kind of magical, because the whole point of homework was to train you in being an accountant for 40 years and sitting on a desk with a visor and doing all that shit, which doesn't have to happen anymore.
Now it's like, okay, what's a great accountant? The person who gives me good advice on how to deal with my taxes, the person who gives me good advice on where I should put my assets or how to do my whatever funky discounting of future leases or whatever.
It's all higher cognitive type stuff and the nice thing about the AI is that, so far as you go up, if you've played with these tools, the higher level you go, the smarter the robot gets.
They really will kind of go up there with you to the point where it's kind of magical. You can have conversations that you wouldn't normally have, and they'll pull data and explore it and that kind of stuff. And I think that you will get more of the supercharged analysts if that makes sense, and a more efficient allocation of labor.
Net-net, the macro question about labor is a huge open question, which is bigger than hedge funds.
It's like the old classic thing of, okay, imagine you take an economy of a hundred dollars and you automate 90% of the jobs, but all of that goes to profit to one person who has a very high savings rate.
What happens to your GDP? It goes crash, right? And so how do you sustain growth in an era of automation and lower wages?
You need debt. And so you have to lever people up to continue to sustain growth. And we've already done that for 40 years, right? And the question is, how bad does that get in the next 40 years?
That's the thing I worry a little bit about, which is like radical inequality. We're not talking like, oh, billionaires. We're talking like Elon has 10 kids and they're all trillionaires.
You know what I mean? Like that's a weird world to live in. It might be pretty unstable when you've automated out every McDonald's worker. You know what I mean?
There's a lot of people who have time on their hands now who might get up to trouble. How we deal with that as a society, I don't know. But I think corporate-wise, like white-collar jobs, that kind of stuff, I think it'll feel pretty natural.
I think it'll feel like the internet. It'll be a little bit faster and a little more disruptive. But the person who's like the worst analyst at whatever, like Citi Banking, doesn't want to be a banker anyway.
They shouldn't be a banker. They should do something else. And that's kind of what the economy is.
This whole where are all the farmers? Well, they're too busy driving Ubers to farm your corn. It's probably a good thing.
James:
Yeah, totally. And look, I think it can go in that direction. However, personally, I have faith that over time, tech transitions tend to be fairly democratic or egalitarian.
Well, for some time, you have the entire golden age of railroads and like Gilded Age and stuff like that. But usually, it does democratize over time and things like that.
And is it a good thing that we no longer have calculators? Not calculators, meaning the machines. I mean calculators, the job where it's usually just rooms full of people doing tables of calculations or whatever.
It's probably a good thing. It's like, was it a deeply fulfilling, happy job in terms of life or whatever? It's like, it's a job.
But if they can be opened up to be able to be doing other things or pursuing different passions because like you're saying—it's like, it's so easy to code for various things, if you also have LLMs and stuff helping you and these other things…
Is it a bad thing that more people have access to tools and other stuff to do more stuff? I definitely don't think it's a bad thing. And that's sort of technology progress writ large with everything.
It's like, if you go back to the people who coded in COBOL, it's like, we definitely wouldn't have the internet that we have today or the products that we have today if all of it was just like the grumpy old guys that coded in COBOL and no one else does it.
Alex:
Exactly.
Some final thoughts against doomerism
James:
Well, I think that's pretty much almost all of my questions here. Is there anything else in terms of last thoughts or whatever that you want to jump in with or something I didn't ask about that I should have?
Alex:
No, I think I'll just make a two-minute case against doomerism while we're here.
So I'm kind of active on the internet. You know, I was trying to fight 1047 in California. Like, I think anyone who works with machines has to have respect for them and the power and the ways they can go wrong and all that stuff.
And, you know, I think when we look at the conversation around AI, it's way too focused on the capabilities. It's kind of inherent fear of something that's smarter than you or something that's, you know, potentially going to be not trustworthy.
It's ironic how many people that make that argument eat meat. You know what I mean? Because they've already established some sort of hierarchy of consciousness where it's okay to be mean to someone who's, you know, whatever, but okay, fine.
I'm much more of a non-zero sum kind of person. Like if you look at the arc, there's a book called Non-Zero that's on this, but if you look at the arc of history, in general, when you play less non-zero sum games, everyone gets better off, you know, less money spent fighting, more money spent, you know, growing and kind of transacting.
And I think if you are worried about AI, we should not make it harder to make them smarter, at least not yet.
We should make it harder to cause chaos. Just think about how intelligence might translate into power, which that power might hurt people, and focus on the power.
And so the most obvious case is like bio. So you know, I put this Manifold market up and I was like, okay, if we do get doomed, what's the most way, easy way we're going to all die? And like, by far the most popular answer is, you know, bio risk.
That's not really AI risk. That's just bio risk. Like maybe we shouldn't be training, you know, viruses to be really deadly. I don't know. Like that's a side thing. Yeah, if you have a lot of that and AI wakes up, bad outcome.
But things like how AI requires money, do they have property rights? How can AI or robots hurt humans? People put cameras on stop signs and for traffic enforcement. And for the first time, you can get a civil penalty from a robot. Well, it’s a bit of a weird slippery slope. What happens if you put a gun on top of the robot? Not really, but there are guns on robot dogs now.
Now on the other hand, all these AI doomers, me as well, get so worked up about every new model. Look, if you work in this field, you see how long this stuff takes.
So, what you should really worry about is not aligning the machine perfectly or preventing it from being smart… But preventing it from acquiring power that might actually be hurtful to people.
And that's true of humans too. That's why we have a legal system, right? So, I'm very big in terms of personal accountability. Like you know, if you do something crazy with a model, you should get kind of strung up or whatever.
But I really, really don't think the right answer is to kill the goose of like making something magically intelligent. Like that seems like a very Luddite kind of naive short-term thinking. And when you put it in the context, not just of China, but every major country in the world, like there's no way we're going to be able to stop all of this stuff at once.
Like there's just no incentive compatibility whatsoever. And you know, given we're entering a world of more conflict and great power kind of standoff, it just seems laughable.
And then you see these people arguing themselves into knots about how, you know, Xi’s really excited about killing AI. And meanwhile, he's the one with the robot dogs. With guns. So, it just makes no sense.
But you know, that's my kind of, well, we have two minutes, my last plea, which is like, don't mess with the science. Don't mess with the capabilities. Don't mess with this magical thing, which is we took sand and now it thinks and talks, and you know, does all this magical stuff.
But don't put the gun in the sand's hand. You know, it's very simple. Just think about that and be vigilant about it. So, a little bit off-topic, but that's kind of my hobby horse lately.
James:
No, I think that's great. And I think just, I agree with you in terms of broad philosophy, but just even summarizing one of the things that you said…
Like someone has to put the gun in that hand, right? It's not going to be another AI. It's going to be a human that has decided to do this and then let the thing run wild.
And at that point, it's not the AI's fault, right?
You just, it's just like, you know, if someone took a tractor and like ran over people, it's not the tractor's fault that ran over people, it's the person driving it.
Alex:
“I put it on full drive, I locked the steering wheel, I pointed it at, you know, name your whatever sensitive area that I won't even mention. And oh my God, terrible things happened.”
It's like, well, who's responsible for that? Is the tractor responsible for that? No, like the human is.
James:
Yeah, totally. I think that's a great note to end on.
And yeah, down to Doomerism, and hopefully we keep going in terms of this stuff and do so responsibly.
Alex:
Awesome.
Thanks for reading!
I hope you enjoyed this interview.
Once again, this is a preview of the type of content in my book.
If you’d like to learn more about AI’s past, present, and future in an easy to understand way, follow updates on the book’s release below!
Share this post